How to leverage Big Data and Machine Learning for business insights
Big data and Machine Learning are hot topics of articles all over tech blogs. The reason is that businesses can receive handy insights from the data generated. The main tools for that are machine learning algorithms for Big data analytics. But how to leverage Machine Learning with Big data to analyze user-generated data? Let’s start with the basics.
What is Big data?
Big data means significant amounts of information gathered, analyzed, and implemented into the business. The “Big data” concept emerged as a culmination of the data science developments of the past 60 years.
How to understand what data could be useful for business insights and what data isn’t? To find this out, you need to consider the following data types:
- Data submitted. When the User creates an account on the website, subscribes to an email newsletter, or performs payments, for example.
- Data is a result of other activities. Web behavior in general and interact with ad content in particular.
Data Mining and further Data Analytics are the heart of Big data solutions. Data Mining stands for collecting data from various sources, while Data Analytics is making sense of it. Sorted and analyzed data can uncover hidden patterns and insights for every industry. How do you make sense of the data? It takes more than to set up a DMP (Data Management Platform) and program a couple of filters to make the incoming information useful. Here’s where Machine Learning comes in.
What is Machine Learning (ML)?
Machine Learning processes data by decision-making algorithms to improve operations.
Usually, machine learning algorithms label the incoming data and recognize patterns in it. Then, the ML model translates patterns into insights for business operations. ML algorithms are also used to automate certain aspects of the decision-making process.
What is Machine Learning in Big data?
ML algorithms are useful for data collection, analysis, and integration. Small businesses with small incoming information do not need machine learning.
But, ML algorithms are a must for large organizations that generate tons of data.
Machine learning algorithms can be applied to every element of Big data operation, including:
- Data Labeling and Segmentation
- Data Analytics
- Scenario Simulation
Let’s look at how businesses use Machine Learning for Big Data analytics.
Machine Learning and Big data use cases
To give you an idea of how businesses combine both technologies, we gathered examples of big data and machine learning projects below.
Market Research & Target Audience Segmentation
Knowing your audience is one of the critical elements of a successful business. But to make a market & audience research, one needs more than surface observations and wild guesses. Machine learning algorithms study the market and help you to understand your target audience.
By using a combination of supervised and unsupervised machine learning algorithms you can find out:
- A portrait of your target audience
- Patterns of their behavior
- Their preferences
This technique is popular in Media & Entertainment, Advertising, eCommerce, and other industries.
To find out more about ML and Big data, watch the video.
Source: Columbia Business School
User Modeling
User Modeling is a continuation and elaboration on Target Audience Segmentation. It takes a deep dive inside the user behavior and forms a detailed portrait of a particular segment. By using machine learning for big data analytics, you can predict the behavior of users and make intelligent business decisions.
Facebook has one of the most sophisticated user modeling systems. The system constructs a detailed portrait of the User to suggest new contacts, pages, ads, communities, and also ad content.
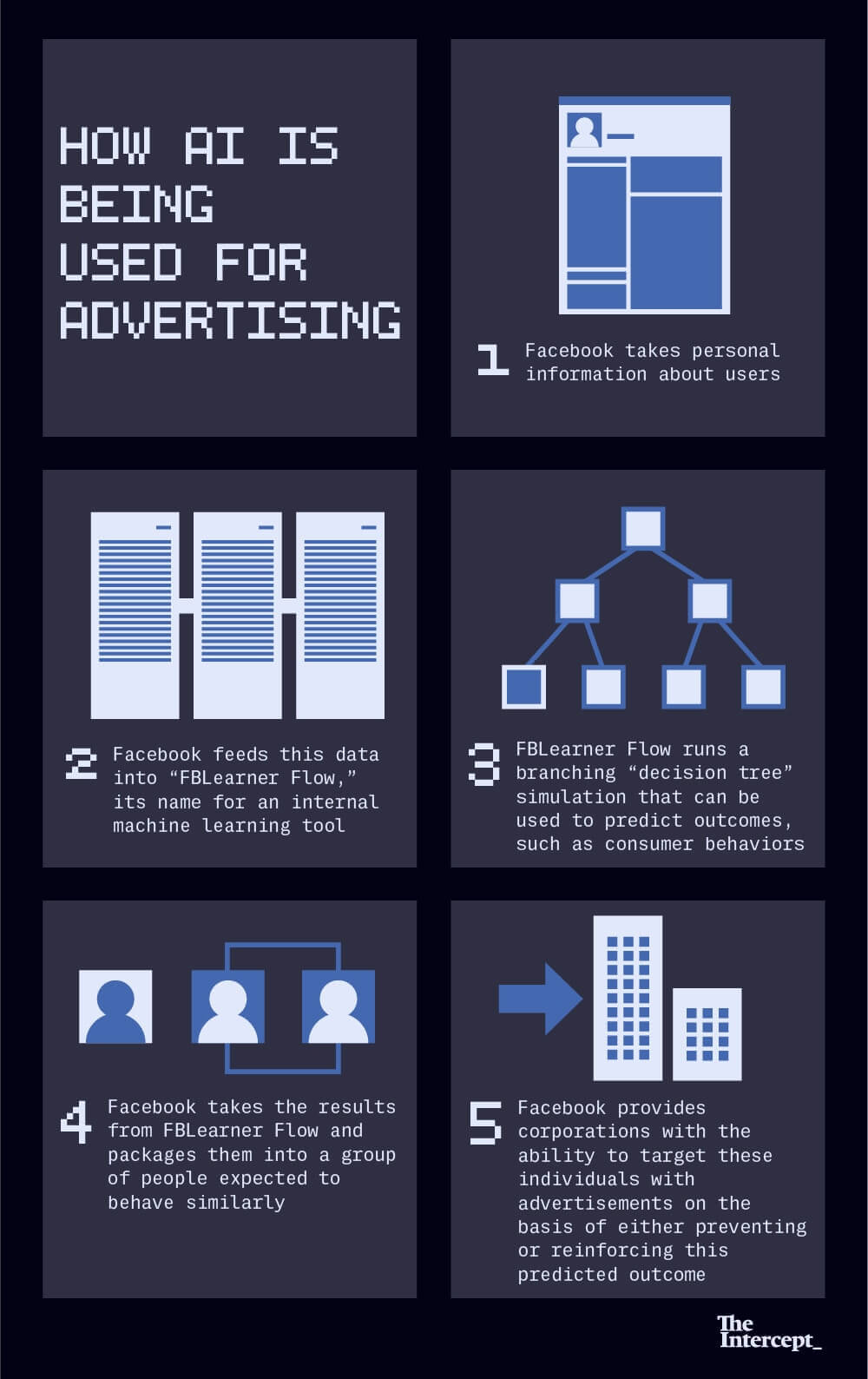
[Source]
Recommendation engines
Ever wondered how Netflix makes on-point suggestions or Amazon shows relevant products from the get-go? That’s because of recommender systems. A recommendation engine is one of the best Big data Machine Learning examples. Such systems can provide a handy suggestion on what types of products are “bought together.” Moreover, they point out the content that might also be interesting to the User who read a particular article.
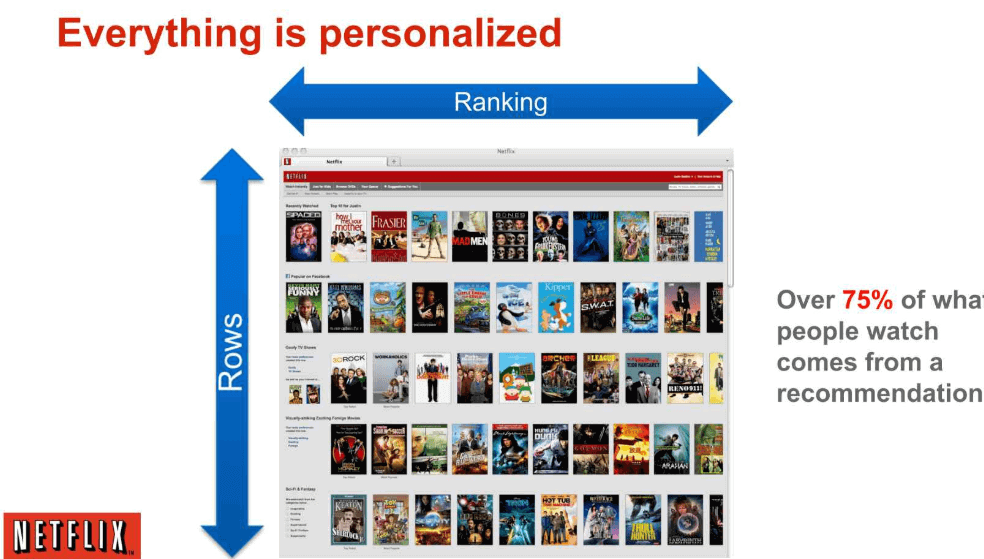
[Source]
Based on a combination of context and user behavior prediction, the recommendation engine can:
- Play on the engagement of the User
- Shape his experience according to his expressed preferences and behavior on-site.
Recommendation engines apply extensive content-based data filtering to extract insights. As a result, the system learns from the User’s preferences and tendencies.
Predictive Analytics
Knowing what the customer needs is one of the foundational elements of retail. That’s market basket analysis in action. Big data allows calculating the probabilities of various outcomes and decisions with a small margin of error.
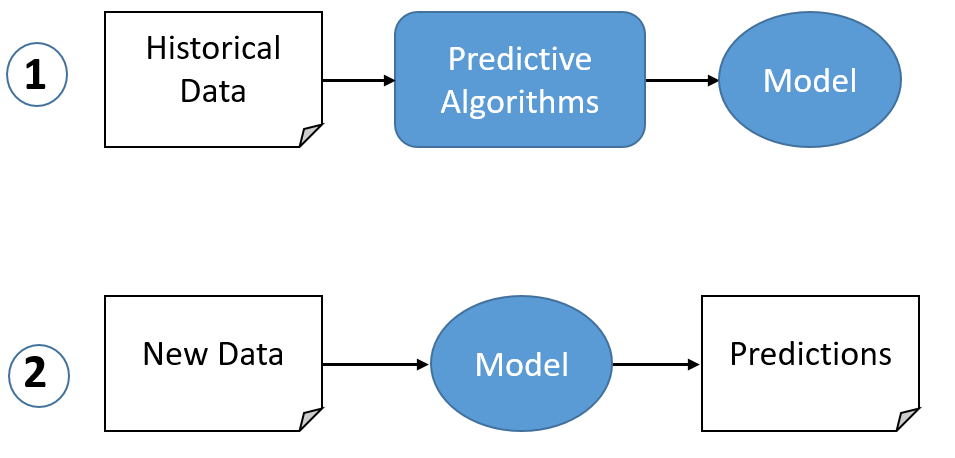
[Source]
Predictive Analytics is useful for:
- Suggesting extra products on eCommerce platforms
- Assessing the possibility of fraudulent activity in ad tech projects
- Calculating the probabilities of treatment efficiency for specific patients in healthcare
One example is eBay’s system that reminds about abandoned purchases, hot deals, or incoming auctions.
Ad Fraud, eCommerce Fraud
Ad Fraud is one of the biggest problems of the Ad Tech industry. The statistics claim that from 10% to 30% of activity in advertising is fraudulent.
Machine Learning algorithms help to fight that by:
- Recognizing the patterns in Big data
- Assessing their credibility
- Blocking them out of the system before the bots or insincere users take over and trash the place
Machine learning algorithms watch ad track activity and block the sources of fraud.
Download Free E-book with DevOps Checklist
Download NowChatbots
Conversational User Interfaces or chatbots are the most use case of Big data & machine learning. By leveraging machine learning algorithms, a chatbot can adapt to a particular customer’s preferences after many interactions
The most well-known AI Assistants are Amazon’s Alexa and Apple’s Siri.
To find out, how does Alexa uses ML algorithms, watch the video.
[Source: Data Science Foundation]
In Conclusion
Big data is an exciting technology with the potential to uncover hidden patterns for more effective solutions. The way it transforms various industries is fascinating. Big data has a positive impact on business operations. Machine learning eliminates routine operations with minimum supervision from humans.
Both Big data and Machine Learning have many use cases in business, from analyzing and predicting user behaviors to learning their preferences. If you have selected the use case of Big data Machine Learning for your business, do not hesitate to hire us for ML development services.