What is Big Data Analytics? Definition, Types, Software, and Use Cases
You can have all the data in the world, but if you don’t know how to use it for your business benefit, there’s no point in sitting on that raw information and expect good things to happen. The solution – Big Data Analytics – helps to gain valuable insights to give you the opportunity to make business decisions more effectively.
In a way, data analytics is the crossroads of the business operations. It is the vantage point where you can watch the streams and note the patterns.
But first – let’s explain the basics.
What is Data Analytics?
The term “Data Analytics” describes a series of techniques aimed at extracting relevant and valuable information from extensive and diverse sets of unstructured data collection from different sources and varying in sizes.
Here’s what you need to understand about data – everything on the internet can be its source. For example:
- content preferences
- different types of interactions with certain kinds of content or ads
- use of certain features in the applications
- search requests
- browsing activity
- online purchases
This data is analyzed and integrated into a bigger context to amplify business operation and make it as effective as possible.
Raw data is like a diamond in the rough. Data Mining takes the rough part, and then Data Analytics provides the polish. That’s the general description of what Big Data Analytics is doing. In fact, data mining gets “unrefined” information “from the bowels”, and then it needs to be finalized depending on the tasks. From that moment on, the process of analyzing raw data goes on.
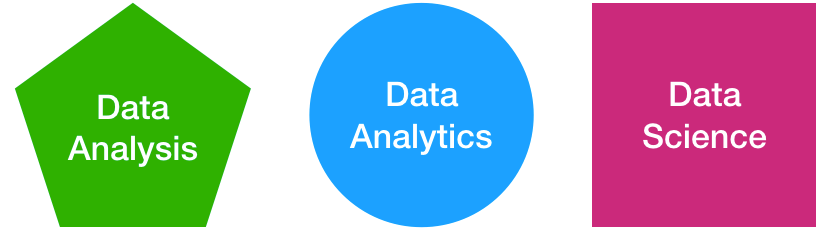
Data Analysis vs. Data Analytics vs. Data Science
Many terms sound the same, but they are different in reality. In case you are confused about what is the difference between data science, analytics, and data analysis, it’s easy to distinguish:
-
The data analysis primarily focuses on processes and functions
-
The data analytics deal with information, dashboards, and reporting
-
The data science includes data analysis but also has elements of data cleaning and preparation for further investigation (from data mining)
Role of Data Analyst in the Business
Data Analysts are the specialists who control the data flows and make sense of the data using specific software.
Basic data analytics operations don’t require specialized personnel to handle the process (usually it can take care of by stand-alone software), but in the case of Big Data analytics, you do need qualified Data Business Analysts.
The purpose of a Data Analyst is to
- Study the information
- Clean it from noise
- Assess the quality of data and its sources
- Develop the scenarios for automation and machine learning
- Oversee the proceedings
Use of Big Data in Data Analytics
You don’t need Big Data for Data Analytics since the latter is about analyzing whatever information you have. However, why is big data important? Because it can be a deeper and more fulfilling source of insights, which is especially useful in the case of prediction and prescription analytics.
Data Analytics is all about making sense of information for your business operation and making use of it in the context of your chosen course of action.
It is essential to understand what kind of statistical analysis needs to be applied to make the most of available information and turn a pile of historical data into a legitimate strategic advantage.
There are four big categories of Data Analytics operation. Let’s look at them one by one.
Different Types of Data Analytics
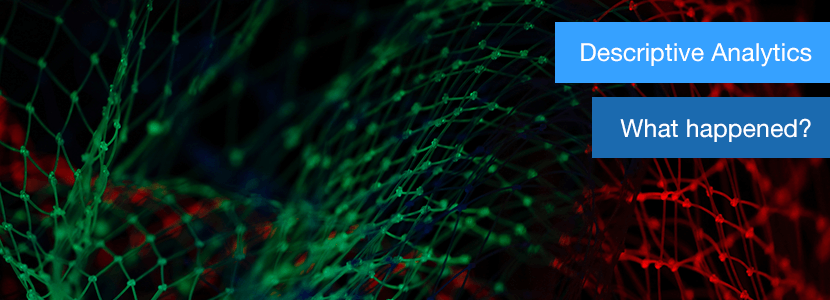
Descriptive Analytics – What Happened?
The purpose of descriptive analytics is to show the layers of available information and present it in a digestible and coherent form. It is the most basic type of data analytics, and it forms the backbone for the other models.
Descriptive analytics is used to understand the big picture of the company’s process from multiple standpoints. In short – it is
- What is going on?
- How is it going on?
- Is it any good for business within a selected period?
Because descriptive analytics are so basic, this type is used throughout industries from marketing and ecommerce to banking and healthcare (and all the other.) One of the most prominent descriptive analytics tools is Google Analytics.
From the technical standpoint, the descriptive operation can be explained as an elaborate “summarizing.” The algorithms process the datasets and arrange them according to the found patterns and defined settings and then present it in a comprehensive form.
For example, you have the results of the marketing campaign for a certain period. In this case, descriptive analytics shows the following stats of interacting with content:
- Who (user ID);
- Circumstances (source – direct, referral, organic);
- When (date);
- How long (session time).
The insights help to adjust the campaign and focus it on more relevant and active segments of the target audience.
Descriptive analytics is also used for the optimization of real-time bidding operation in Ad Tech. In this case, the analytics show the effectiveness of spent budgets and shows the correlation between spending and the campaign’s performance. Depending on the model, the efficiency is calculated using goal actions like conversions, clicks, or views.
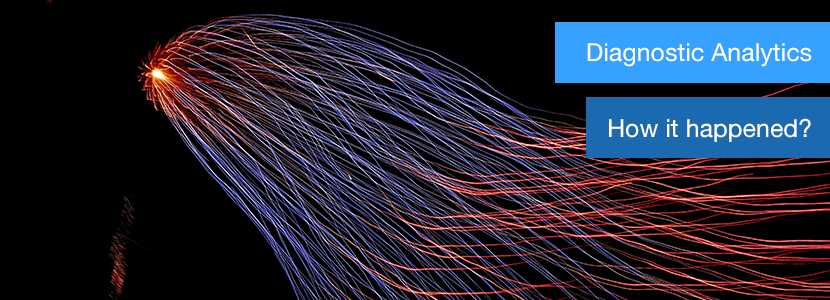
Diagnostic Analytics – How It Happened?
The purpose of diagnostic analytics is to understand:
- why certain things happened
- what caused these turns of events.
Diagnostic analytics is an investigation aimed at studying the effects and developing the right kind of reaction to the situation.
The operation includes the following steps:
- Anomaly Detection. The anomaly is anything that raises the question of its appearance in the analytics, whatever doesn’t fit the norm. It can be a spike of activity when it wasn’t expected or a sudden drop in the subscription rate of your social media page.
- Anomaly Investigation. To do something, you need to understand how it happened. This process includes the identification of sources and finding patterns in the data sources.
- Causal Relationship Determination. After the events that caused anomalies are identified – it is time to connect the dots. This may involve the following practices:
- Probability analysis
- Regression analysis
- Filtering
- Time-series data analytics
Diagnostic Analytics is often used in Human Resources management to determine the qualities and potential of employees or candidates for positions.
It can also apply comparative analysis to determine the best fitting candidate by selected characteristics or to show the trends and patterns in a specific talent pool over multiple categories (such as competence, certification, tenure, etc.)
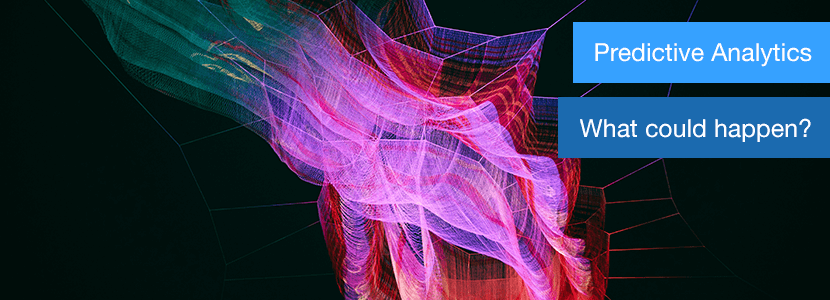
Predictive Analytics – What Could Happen?
As you might’ve guessed from the title – predictive analytics is designed to foresee:
- what the future holds (to a certain degree)
- show a variety of possible outcomes
In business, it’s often much better to be proactive rather than reactive. Therefore, Predictive Analytics helps you to understand how to make successful business decisions that bring value to companies.
How do the Predictive Analytics algorithms work?
- Go through the available data from all relevant sources (for example, it can be one source or a combination of ERP, CRM, HR systems);
- Combine it into one big thing;
- Identify patterns, trends, and anomalies;
- Calculate possible outcomes.
While predictive analytics estimates the possibilities of certain outcomes, it doesn’t mean these predictions are a sure thing. However, armed with these insights, you can make wiser decisions.
Have a Project In Mind?
Estimate Its CostsApplication areas of Predictive Analytics:
- Marketing – to determine trends and potential of particular courses of action. For example, to define the content strategy and types of content more likely to hit the right chord with the audiences;
- Ecommerce / Retail – to identify trends in customer’s purchase activities and operate product inventory accordingly.
- Stock exchanges – to predict the trends of the market and the possibilities of changes in various scenarios.
- Healthcare – to understand possible outcomes of disease outbreak and its treatment methodology. It is used for scenario simulation studies and training.
- Sports – for predicting game results and keeping track of betting;
- Construction – to assess structures and material use;
- Accounting – for calculating probabilities of certain scenarios, assessing current tendencies, and providing several options for decision making.
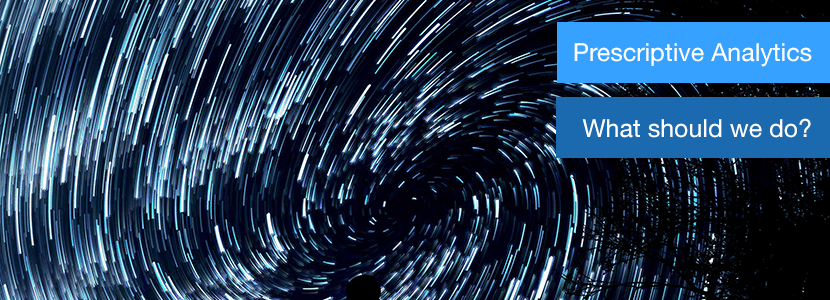
Prescriptive Analytics – What Should We Do?
Not to confuse prescriptive and predictive analytics:
- Predictive analytics says what might happen in the future
- Prescriptive analytics is all about what to do in the future
This digging into customer data presents a set of possibilities and opportunities as well as options to consider in various scenarios.
Tech-wise, prescriptive analytics consists of a combination of:
- specific business rules and requirements,
- selection of machine learning algorithms (usually supervised)
- modeling procedures
All this is used to calculate as many options as possible and assess their probabilities.
Then you can turn to predictive analytics and look for further outcomes (if necessary). It is commonly used for the following activities:
- Optimization procedures;
- Campaign management;
- Budget management;
- Content scheduling;
- Content optimization;
- Product inventory management.
Prescriptive analytics is used in a variety of industries. Usually, it is used to provide an additional perspective into the data and give more options to consider upon taking action, for example:
- Marketing – for campaign planning and adjustment;
- Healthcare – for treatment planning and management;
- E-commerce / Retail – in inventory management and customer relations;
- Stock Exchanges – in developing operating procedures;
- Construction – to simulate scenarios and better resource management.
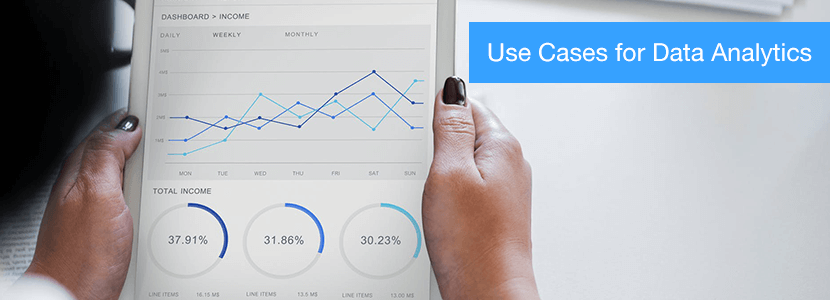
Big Data Analytics Use Cases
Now let’s look at the fields where data analytics makes a critical contribution.
Sales and Operations Planning Tools
Sales and operations planning tools are something like a unified dashboard from which you can perform all actions. In other words, it is a tight-knit system that uses data analytics on a full scale.
As such, S&OP tools are using a combination of all four types of data analytics and related tools to show and interact with the available information from multiple perspectives.
These tools are aimed specifically at developing overarching plans with every single element of operation past, present, or future is taken into consideration to create a strategy as precise and flexible as possible.
The most prominent examples are the Manhattan S&OP and Kinxaxis Rapid Response S&OP. However, it should be noted that there are also custom solutions tailor-made for the specific business operation.
Download Free E-book with DevOps Checklist
Download NowRecommendation Engines
Internal and external recommender engines and content aggregators are some of the purest representations of data analytics on a consumer level.
The mechanics behind it is simple:
- The user has some preferences and requirements, noted by the system.
- Web crawling or internal search tools for relevant matches based on user preferences.
- If there is a match, it’s included in the options.
There are two types of user preferences that affect the selection:
- Direct feedback via ratings;
- Indirect via interacting with the specific content from the various sites.
As a result of this, the user gets the content s/he will most likely interact with offered.
One of the most prominent examples of this approach is used by Amazon and Netflix search engines. Both of them are using extensive user history and behavior (preferences, search queries, watch time) to calculate the relevancy of the suggestions of the particular products.
Also, Google Search Engine personalization features enable more relevant results based on expressed user preferences.
Customer Modelling / Audience Segmentation
The customer is always on the front stage. One of the most common usages of data analytics is aimed at:
- Defining and describing customers;
- Recognizing distinct audience segments;
- Calculating their possible courses of actions in certain scenarios.
Since the clearly defined target audience is the key for a successful business operation – user modelling is widely used in a variety of industries, most prominently in digital advertising and e-commerce.
How does it work? Every piece of information that the user produces keeps some insight that helps to understand what kind of product or content he might be interested in.
This information helps to construct a big picture of:
- Who is your target audience;
- Which segments are the most active;
- What kind of content or product can be targeted towards which of the audience segments;
Amazon is good at defining audience segments and relevant products to the particular customer (which helps it to earn a lot of money, too.)
We have our case study regarding user modeling and segmentation with the Eco project. In that case, we did a cross-platform analytics solution that studied the patterns of product use in order to determine audience segments and improve user experience across the board.
Market Research / Content Research
Knowledge is half of the battle won and nothing can do it better than a well-tuned data analytics system.
Just as you can use data analytics algorithms to determine and thoroughly describe your customer, you can also use similar tools to describe the environment around you and get to know better what the current market situation is and what kind of action should be taken to make the most out of it.
Fraud Prevention
Powers of hindsight and foresight can help to expose fraudulent activities and provide a comprehensive picture.
The majority of fraudulent online activities are made with the assistance of automated mechanisms. The thing with automated mechanisms is that they work in patterns and patterns are something that can be extracted out of the data.
This information can be integrated into a fraud detecting system. Such approaches are used to filter out spam and detect unlawful activities with doubtful accounts or treacherous intentions.
Price Optimization
One of the critical factors in maintaining competitiveness on the market in e-commerce and retail is having more attractive prices than the competition.
In this case, the role of data analytics is simple – to watch the competition and adjust the prices of the product inventory accordingly.
The system is organized around a couple of mechanisms:
- Crawler tool that checks the prices on the competitor’s marketplaces;
- Price comparison tool which includes additional fees such as shipping and taxes;
- Price adjustment tool that automatically changes the cost of a particular product.
To manage discounts or special offer campaigns, one can also use these tools.
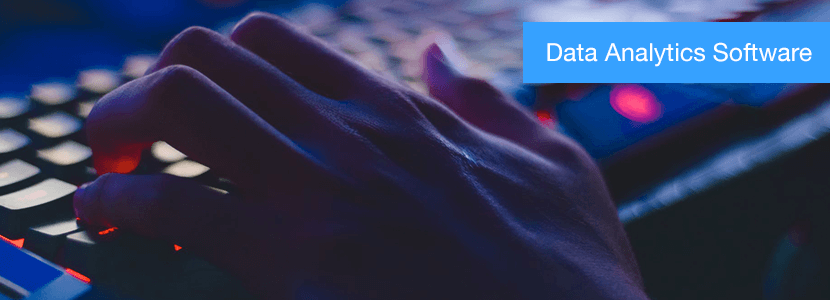
Big Data Tools: Data Analytics Software
In addition to custom solutions, there are several useful ready-made data analytics tools that you can fit into your business operation.
Basic
- Excel Spreadsheet – the most basic tool for data analytics. It can be done manually and show enough information to understand what is going on in general terms.
- Google Analytics is a standard descriptive analytics tool that provides information about website traffic, source and basic stats on user behavior. Can be used for further visualization via Data Studio.
- Zoho Reports is a part-descriptive-analytics part-task-management tool. Useful for project reporting and tracking progress. Works well to assess campaign performance results.
Sophisticated
- Tableau Desktop – with this tool you can make any scope of data comprehensible in the form of graphs and tables. Good for putting things into perspective. Easy to use, light on the pocket.
- The demo analytics tool is good for medium-sized operations to gather and analyze data from their own large networks. In addition to visualization, it is capable of assessing probabilities of different scenarios and proposing better fitting courses of action.
- Style Scope is useful for teamwork and planning. It maps data from a different source on one map and in the process unlocks its hidden possibilities.
Heavy Artillery
- Microsoft Power BI can consolidate incoming data from multiple sources, extract insights, assess probabilities of various turns of events, and put them into a broader context with a detailed breakdown of possible options. In other words, it turns Jackson Pollock’s painting into Piet Mondrian’s grid.
- Looker is a multi-headed beast of a tool. With its help, you can combine data from multiple sources – track overall progress, break it down to the element, extract insights, and calculate possibilities – all in one convenient dashboard.
- SAP ERP is primarily used for sales management and resource planning. With its help, you can map out the goals, combine information, assess performance and possibilities, and decide what to do next.
In Conclusion
Here we figured out – what is data analytics? These days, data analytics is one of the key technologies in the business operation. Data mining provides the information, and Data Analytics helps to gain useful insights from that information to integrate them into the business process and enjoy the benefits.
So take advantage of data analytics as a compass to navigate in the sea of information.
Read also: Big Data in Customer Analytics
Looking for a data analytics solution? Let's create a custom one.
Write to us