Data Mining: The Definitive Guide to Techniques, Examples, and Challenges
We live in the age of massive data production. If you think about it – pretty much every gadget or service we are using creates a lot of information (for example, Facebook processes around 500+ terabytes of data each day). All this data goes straight back to the product owners, which they can use to make a better product. This process of gathering data and making sense of it is called Data Mining.
However, this process is not as simple as it seems. It is essential to understand the hows, whats, and whys of data mining to use it to its maximum effect.
What is Data Mining?
Data mining is the process of sorting out the data to find something worthwhile. If being exact, mining is what kick-starts the principle “work smarter not harder.”
At a smaller scale, mining is any activity that involves gathering data in one place in some structure. For example, putting together an Excel Spreadsheet or summarizing the main points of some text.
Data mining is all about:
- processing data;
- extracting valuable and relevant insights out of it.
Purpose of Data Mining
There are many purposes data mining can be used for. The data can be used for:
- detecting trends;
- predicting various outcomes;
- modeling target audience;
- gathering information about the product/service use;
Data mining helps to understand certain aspects of customer behavior. This knowledge allows companies to adapt accordingly and offer the best possible services.
Big Data vs. Data Mining
Let’s put this thing straight:
- Big Data is the big picture, the “what?” of it all.
- Data Mining is a close-up on the incoming information – can be summarized as “how?” or “why?”
Now let’s look at the ins and outs of Data Mining operations.
How Does Data Mining Work?
Stage-wise, data mining operation consists of the following elements:
- Building target datasets by selecting what kind of data you need;
- Preprocessing is the groundwork for the subsequent operations. This process is also known as data exploration.
- Preparing the data – a creation of the segmenting rules, cleaning data from noise, handling missing values, performing anomaly checks, and other operations. This stage may also include further data exploration.
- Actual data mining starts when a combination of machine learning algorithms gets to work.
Data Mining Machine Learning Algorithms
Overall, there are the following types of machine learning algorithms at play:
- Supervised machine learning algorithms are used for sorting out structured data:
- Classification is used to generalize known patterns. This is then applied to the new information (for example, to classify email letter as spam);
- Regression is used to predict certain values (usually prices, temperatures, or rates);
- Normalization is used to flatten the independent variables of data sets and restructure data into a more cohesive form.
- Unsupervised machine learning algorithms are used for the exploration of unlabeled data:
- Clustering is used to detect distinct patterns (AKA groups AKA structures
- Association rule learning is used to identify the relationship between the variables of the data set. For example, what kind of actions are performed most frequently;
- Summarization is used for visualization and reporting purposes;
- Semi-supervised ML algorithms are a combination of the aforementioned methodologies;
- Neural Networks – these are complex systems used for more intricate operations.
Now let’s take a look at the industries where mining is applied.
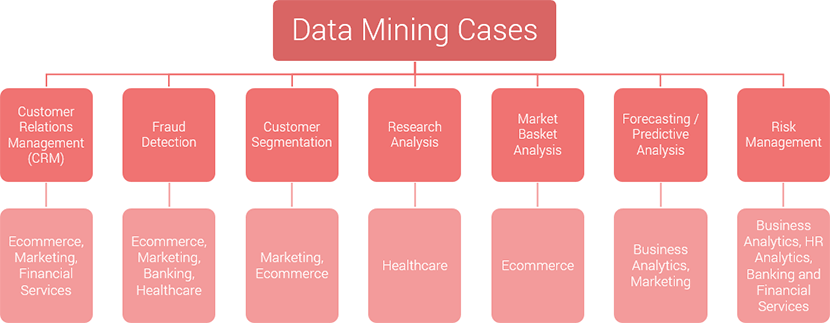
Examples of Data Mining in business
Marketing, eCommerce, Financial Services – Customer Relationship Management
All industries can benefit from CRM systems that are widely used in a variety of industries – from marketing to eCommerce to healthcare and leisure.
The role of data mining in CRM is simple:
- To get insights that will provide a solid ground for attaining and retaining customers
- To adapt services according to the ebbs and flows of the user behavior patterns.
Usually, data mining algorithms are used for two purposes:
- To extract patterns out of data;
- To prepare predictions regarding certain processes;
Customer Relationship Management relies on processing large quantities of data in order to deliver the best service based on solid facts. Such CRMs as Salesforce and Hubspot are built around it.
The features include:
- Basket Analysis (tendencies and habits of users);
- Predictive Analytics
- Sales forecasting;
- Audience segmentation;
- Fraud detection;
eCommerce, Marketing, Banking, Healthcare – Fraud Detection
As it was explained in our Ad Fraud piece, fraud is one of the biggest problems of the Internet. Ad Tech suffers from it, eCommerce is heavily affected, banking is terrorized by it.
However, the implementation of data mining can help to deal with fraudulent activity more efficiently. Some patterns can be spotted and subsequently blocked before causing mayhem, and the application of machine learning algorithms helps this process of detection.
Overall, there are two options:
- Supervised learning – when the dataset is labeled either “fraud” or “non-fraud” and algorithm trains to identify one and another. In order to make this approach effective, you need a library of fraud patterns specific to your type of system.
- Unsupervised learning is used to assess actions (ad clicks, payments), which are then compared with the typical scenarios and identified as either fraudulent or not.
Here’s how it works in different industries:
- In Ad Tech, data mining-based fraud detection is centered around unusual and suspicious behavior patterns. This approach is effective against click and traffic fraud.
- In Finance, data mining can help expose reporting manipulations via association rules. Also – predictive models can help handle credit card fraud.
- In Healthcare, data mining can tackle manipulations related to medical insurance fraud.
Marketing, eCommerce – Customer Segmentation
Knowing your target audience is at the center of any business operation. Data mining brings customer segmentation to a completely new level of accuracy and efficiency. Ever wondered how Amazon knows what are you looking for? This is how.
Customer segmentation is equally important for ad tech operation and for eCommerce marketers. Customer’s use of a product or interaction with ad content provides a lot of data. These bits and pieces of data show customers:
- Interests
- Tendencies and preferences
- Needs
- Habits
- General behavior patterns
This allows constructing more precise audience segments based on practical aspects instead of relying on demographic elements. Better segmentation leads to better targeting, and this leads to more conversions which is always a good thing.
You can learn more about it in our article about User Modelling.
Healthcare – Research Analysis
The research analysis is probably the most direct use of data mining operations. Overall, this term covers a wide variety of different processes that are related to the exploration of data and identifying its features.
The research analysis is used to develop solutions and construct narratives out of available data. For example, to build a timeline and progression of a disease outbreak.
The role of data mining in this process is simple:
- Cleaning the volumes of data;
- Processing the datasets;
- Adding the results to the big picture.
The critical technique, in this case, is pattern recognition.
The other use of data mining in research analysis is for visualization purposes. In this case, the tools are used to reiterate the available data into more digesting and presentable forms.
eCommerce – Market Basket Analysis
Modern eCommerce marketing is built around studying the behavior of the users. It is used to improve customer experience and make the most out of every customer. In other words, it uses user experience to perpetuate customer experience via extensive data mining.
Market basket analysis is used:
- To group certain items in specific groups;
- To target them to the users who happened to be purchasing something out of a particular group.
The other element of the equation is differential analysis. It performs a comparison of specific data segments and defines the most effective option — for example, the lowest price in comparison with other marketplaces.
The result gives an insight into customers’ needs and preferences and allows them to adapt the surrounding service to fit it accordingly.
Business Analytics, Marketing – Forecasting / Predictive Analytics
Understanding what the future holds for your business operation is critical for effective management. It is the key to making the right decisions from a long-term perspective.
That’s what Predictive Analytics are for. Viable forecasts of possible outcomes can be realized through combinations of the supervised and unsupervised algorithm. The methods applied are:
- Regression analysis;
- Classification;
- Clustering;
- Association rules.
Here’s how it works: there is a selection of factors critical to your operation. Usually, it includes user-related segmentation data plus performance metrics.
These factors are connected with an ad campaign budget and also goal-related metrics. This allows us to calculate a variety of possible outcomes and plan out the campaign in the most effective way.
Business Analytics, HR analytics – Risk Management
The Decision-making process depends on a clear understanding of possible outcomes. Data mining is often used to perform a risk assessment and predict possible outcomes in various scenarios.
In the case of Business Analytics, this provides an additional layer for understanding the possibilities of different options.
In the case of HR Analytics, risk management is used to assess the suitability of the candidates. Usually, this process is built around specific criteria and grading (soft skills, technical skills, etc.)
This operation is carried out by composing decision trees that include various sequences of actions. In addition, there is a selection of outcomes that may occur upon taking them. Combined they present a comprehensive list of pros and cons for every choice.
Decision tree analysis is also used to assess the cost-benefit ratio.
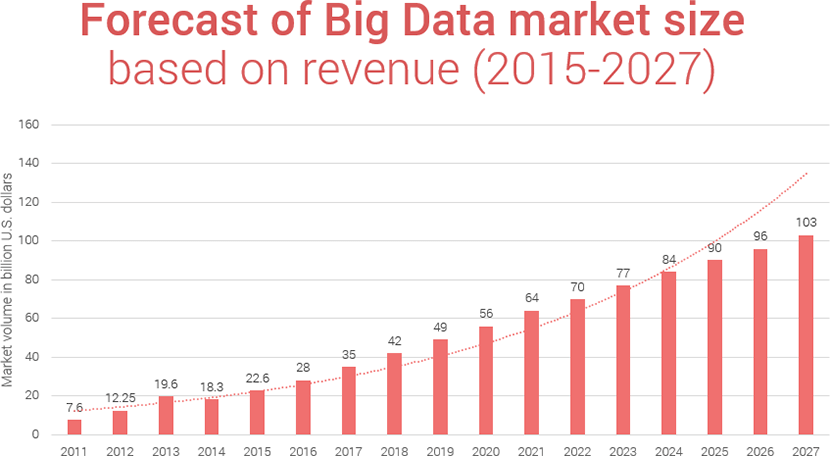
Data Mining Challenges
The scope of Data Sets
While it might seem obvious for big data, but the fact remains – there is too much data. Databases are getting bigger and it is getting harder to get around them in any kind of comprehensive manner.
There is a critical challenge in handling all this data effectively and the challenge itself is threefold:
- Segmenting data – recognizing important elements;
- Filtering the noise – leaving out the noise;
- Activating data – integrating gathered information into the business operation;
Every aspect of this challenge requires the implementation of different machine learning algorithms.
Privacy & Security
Data Mining operation directly deals with personally identifiable information. Because of that, it is fair to say that privacy and security concerns are a big challenge for Data Mining.
It is easy to understand why. Given the history of recent data breaches – there is certain distrust in any data gathering.
In addition to that, there are strict regulations regarding the use of data in the European Union due to GDPR. They turn the data collection operation on its head. Because of that, it is still unclear how to keep the balance between lawfulness and effectiveness in the data-mining operation.
If you think about it, data mining can be considered a form of surveillance. It deals with information about user behavior, consuming habits, interactions with ad content, and so on. This information can be used both for good and bad things. The difference between mining and surveillance lies in the purpose. The ultimate goal of data mining is to make a better customer experience.
Because of that, it is important to keep all the gathered information safe:
- from being stolen;
- from being altered or modified;
- from being accessed without permission.
In order to do that, the following methods are recommended:
- Encryption mechanisms;
- Different levels of access;
- Consistent network security audits;
- Personal responsibility and clearly defined consequences of the perpetration.
Download Free E-book with DevOps Checklist
Download NowData Training Set
To provide a desirable level of efficiency of the algorithm – a training data set must be adequate for the cause. However, that is easier said than done.
There are several reasons for that:
- Dataset is not representative. A good example of this can be rules for diagnosing patients. There must be a wide selection of use cases with different combinations in order to provide the required flexibility. If the rules are based on diagnosing children, the algorithm’s application to adults will be ineffective.
- Boundary cases are lacking. Boundary case means detailed distinction of what is one thing and what is the other. For example, the difference between a table and a chair. In order to differentiate them, the system needs to have a set of properties for both. In addition to that, there must be a list of exceptions.
- Not enough information. In order to attain efficiency, a data mining algorithm needs clearly defined and detailed classes and conditions of objects. Vague descriptions or generalized classification can lead to a significant mess in the data. For example, a definitive set of features that differentiate a dog from a cat. If the attributes are too vague – both will simply end up in the “mammal” category.
Data Accuracy
The other big challenge of data mining is the accuracy of the data itself. In order to be considered worthwhile, gathered data needs to be:
- complete;
- accurate;
- reliable.
These factors contribute to the decision making process.
There are algorithms designed to keep it intact. In the end, the whole thing depends on your understanding of what kind of information you for which kind of operations. This will keep the focus on the essentials.
Data Noise
One of the biggest challenges that come while dealing with Big Data and Data Mining, in particular, is noise.
Data Noise is all the stuff that provides no value for the business operation. As such it must be filtered out so that the primary effort would be concentrated on the valuable data.
To understand what is noise in your case – you need to define what kind of information you need clearly, which forms a basis for the filtering algorithms.
In addition to that, there are two more things to deal with:
- Corrupted attribute values
- Missing attribute values
The thing with both is that these factors affect the quality of the results. Whether it is a prediction or segmenting – the abundance of noise can throw a wrench into an operation.
In case of corrupted values – it all depends on the accuracy of the established rules and the training set. The corrupted values come from inaccuracies in the training set that subsequently cause errors in the actual mining operation. At the same time, values that are worthwhile may be considered as noise and filtered out.
There are times when the attribute values can be missing from the training set and, while the information is there, it might get ignored by the mining algorithm due to being unrecognized.
Both of these issues are handled by unsupervised machine learning algorithms that perform routine checks and reclassifications of the datasets.
What’s Next?
Data Mining is one of the pieces for the bigger picture that can be attained by working with Big Data. It is one of the fundamental techniques of modern business operation. It provides the material that makes possible productive work.
As such, its approaches are continually evolving and getting more efficient in digging out the insights. It is fascinating to see where technology is going.
Does your business need data mining solutions?
Let's discuss the details