Google Cloud Services for Big Data Projects
Google Cloud Platform provides various services for data analysis and Big Data applications. All those services are integrable with other Google Cloud products, and all of them have their pros and cons.
This article will review what services Google Cloud Platform can offer for data and Big Data applications and what those services do. We’ll also check out what benefits and limitations they have, the pricing strategy of each service, and their alternatives.
Cloud PubSub
Cloud PubSub is a message queue broker that allows applications to exchange messages reliably, quickly, and asynchronously. Based on the publish-subscription pattern.
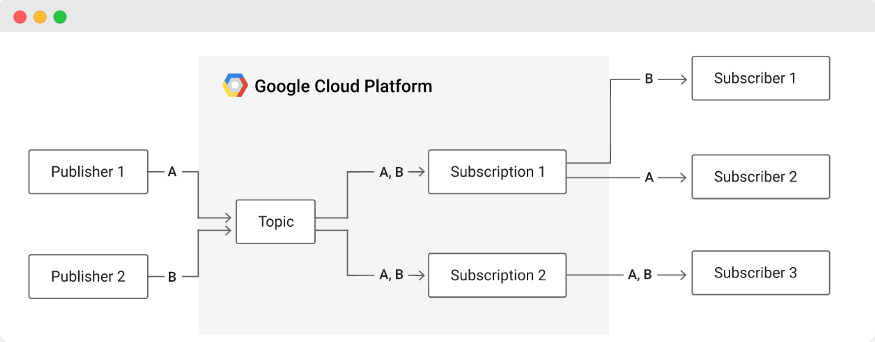
[Visualization of PubSub workflow]
The diagram above describes the basic flow of the PubSub. First, publisher applications publish messages to a PubSub topic. Then the topic sends messages to PubSub subscriptions; the subscriptions store messages; subscriber applications read messages from the subscriptions.
Benefits
- A highly reliable communication layer
- High capacity
Limitations
- 10 MB is the maximum size for one message
- 10 MB is the maximum size for one request, which means if we need to send ten messages per request, the average total length for each notification will be 1 MB.
- The maximum attribute value size is 1 MB
Pricing strategy
You pay for transferred data per GB.
Analogs & alternatives
- Apache Kafka
- RabbitMQ
- Amazon SQS
- Azure Service Bus
- Other Open Source Message Brokers
Google Cloud IoT Core
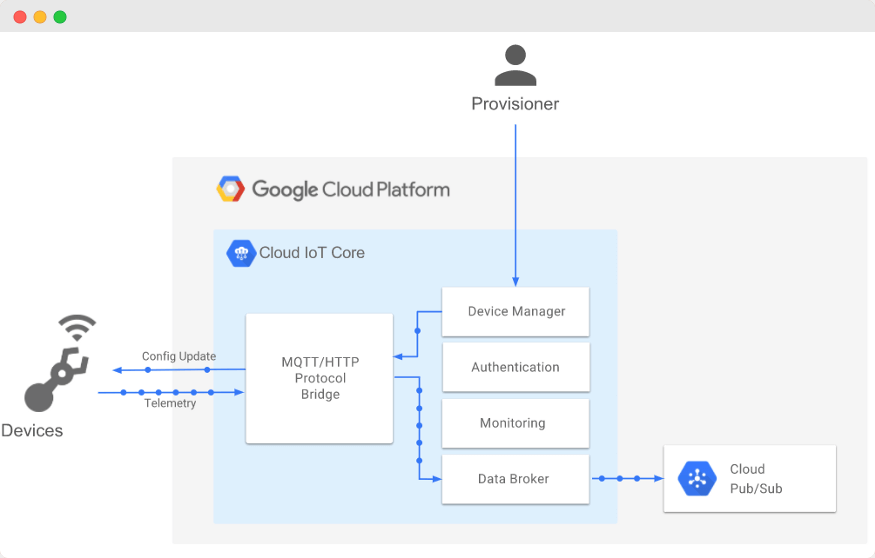
[The architecture of Cloud IoT Core]
Cloud IoT Core is an IoT devices registry. This service allows devices to connect to the Google Cloud Platform, receive messages from other devices, and send messages to those devices. To receive messages from devices, IoT Core uses Google PubSub.
Benefits
- MQTT and HTTPS transfer protocols
- Secure device connection and management
Pricing Strategy
You pay for the data volume that you transfer across this service.
Analogs & alternatives
- AWS IoT Core
- Azure IoT
Cloud Dataproc
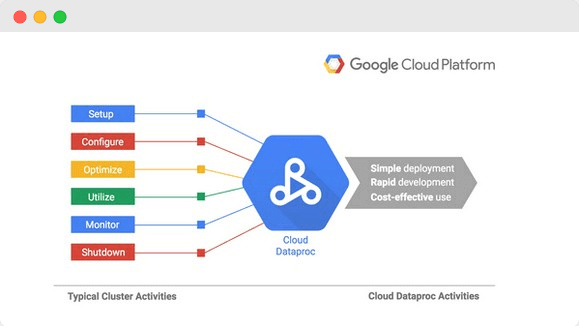
Cloud Dataproc is a faster, easier, and more cost-effective way to run Apache Spark and Apache Hadoop in Google Cloud. Cloud Dataproc is a cloud-native solution covering all operations related to deploying and managing Spark or Hadoop clusters.
In simple terms, with Dataproc, you can create a cluster of instances on Google Cloud Platform, dynamically change the size of the cluster, configure it, and run MapReduce jobs.
Benefits
- Fast deployment
- Fully managed service means you need just the right code, no operation work
- Dynamically resize the cluster
- Auto-Scaling feature
Limitations
- No choice of selecting a specific version of the used framework
- You cannot pause/stop Data Proc Cluster to save money. Only delete the cluster. It’s possible to do via Cloud Composer
- You cannot choose a cluster manager, only YARN
Pricing strategy
You pay for each used instance with some extra payment—Google Cloud Platform bills for each minute when the cluster works.
Analogs & alternatives
- Set-up cluster on virtual machines
- Amazon EMR
- Azure HDInsight
Cloud Dataflow
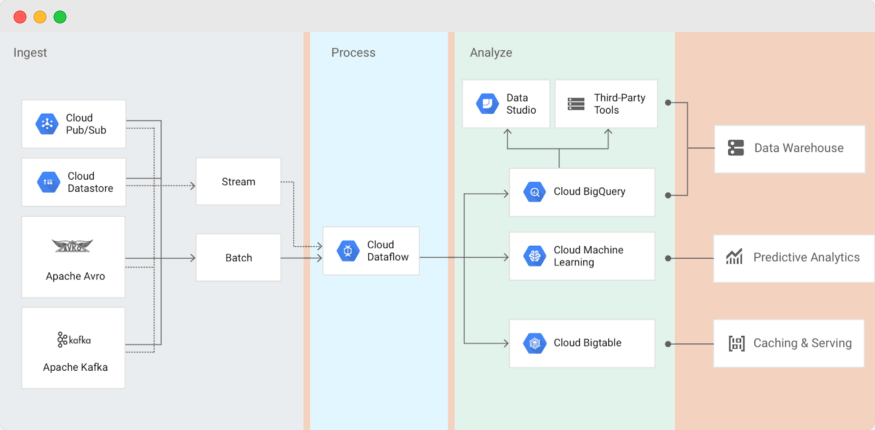
[The place of Cloud Dataflow in a Big Data application on Google Cloud Platform]
Cloud Dataflow is a managed service for developing and executing a wide range of data processing patterns, including ETL, batch, streaming processing, etc. In addition, Dataflow is used for building data pipelines. This service is based on Apache Beam and supports Python and Java jobs.
Benefits
- Combines batch and streaming with a single API
- Speedy deployment
- A fully managed service, no operation work
- Dynamic work rebalancing
- Autoscaling
Limitations
- Based on a single solution, therefore, inherits all limitations of Apache Beam
- The maximum size for a single element value in Streaming Engine is 100 Mb
Pricing strategy
Cloud Dataflow jobs are billed per second, based on the actual use of Cloud Dataflow.
Analogs & alternatives
- Set-up cluster on virtual machines and run Apache Beam via in-built runner
- As far as I know, other cloud providers don’t have analogs.
Google Cloud Dataprep
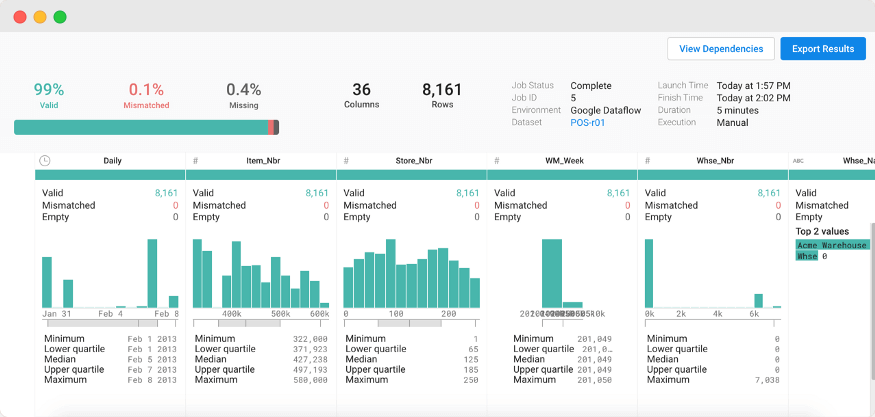
[The interface of Dataprep]
Dataprep is a tool for visualizing, exploring, and preparing data you work with. You can build pipelines to ETL your data for different storage. And do it on a simple and intelligible web interface.
For example, you can use Dataprep to build the ETL pipeline to extract raw data from GCS, clean up this data, transform it to the needed view, and load it into BigQuery. Also, you can schedule a daily/weekly/etc job that will run this pipeline for new raw data.
Benefits
- Simplify building of ETL pipelines
- Provide a clear and helpful web interface
- Automate a lot of manual jobs for data engineers
- Built-in scheduler
- To perform ETL jobs, Dataprep uses Google Dataflow
Limitations
- Works only with BigQuery and GCS
Pricing Strategy
For data storing, you pay for data storage. For executing ETL jobs, you pay for Google Dataflow.
Cloud Composer
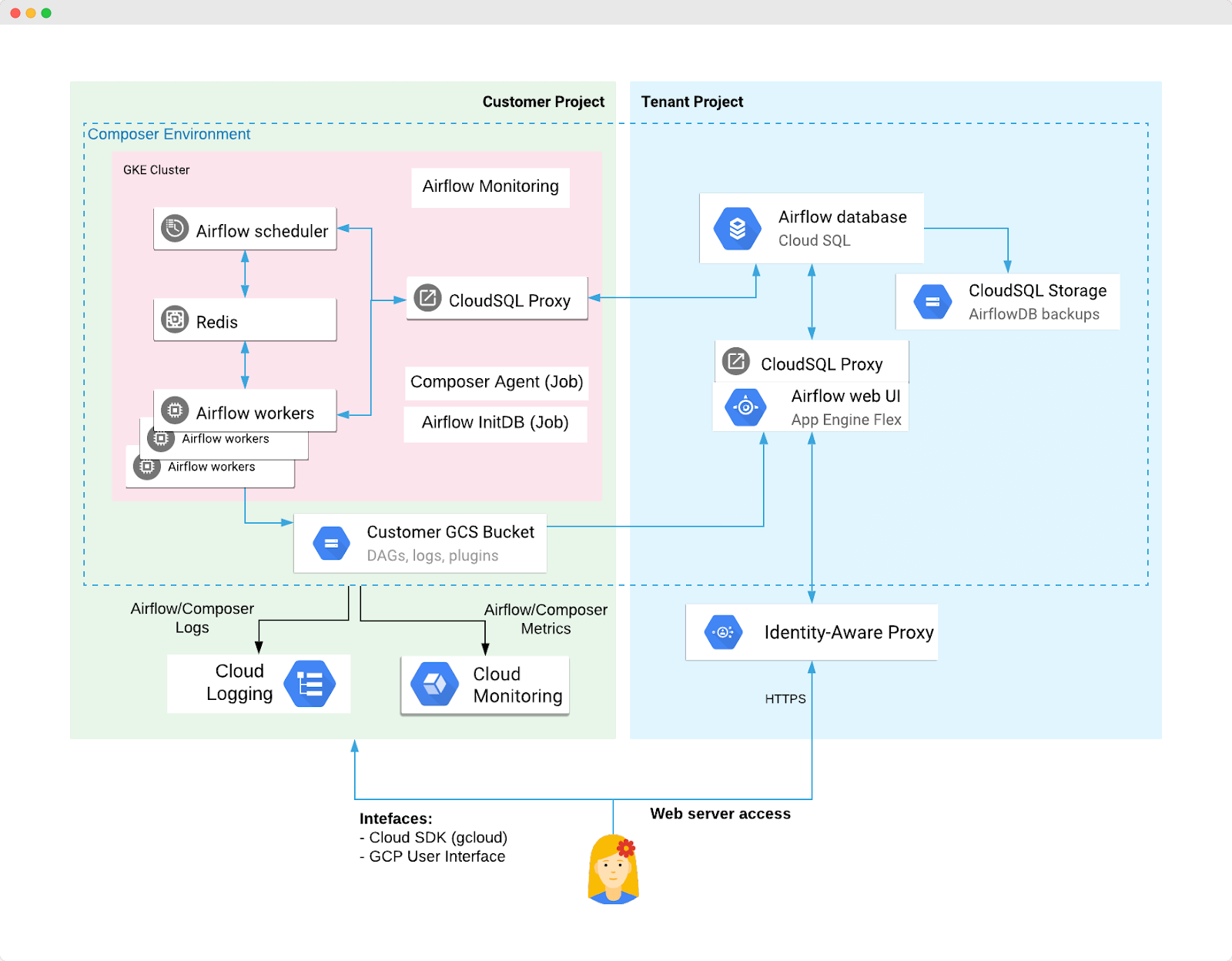
Cloud Composer is a workflow orchestration service to manage data processing. Cloud Composer is a cloud interface for Apache Airflow. Composer automates the ETL jobs. One example is to create a Dataproc cluster, perform transformations on extracted data (via a Dataproc PySpark job), upload the results to BigQuery, and then shut down the Dataproc collection.
Benefits
- Fills the gaps of other Google Cloud Platform solutions, like Dataproc
- Inherits all advantages of Apache Airflow
Limitations
- Provides the Airflow web UI on a public IP address
- Inherits all rules of Apache Airflow
Pricing Strategy
You pay only for resources on which Composer is deployed. But the Composer will be deployed to 3 instances.
Analogs & alternatives
- Custom deployed Apache Airflow
- Other orchestration open source solution
BigQuery
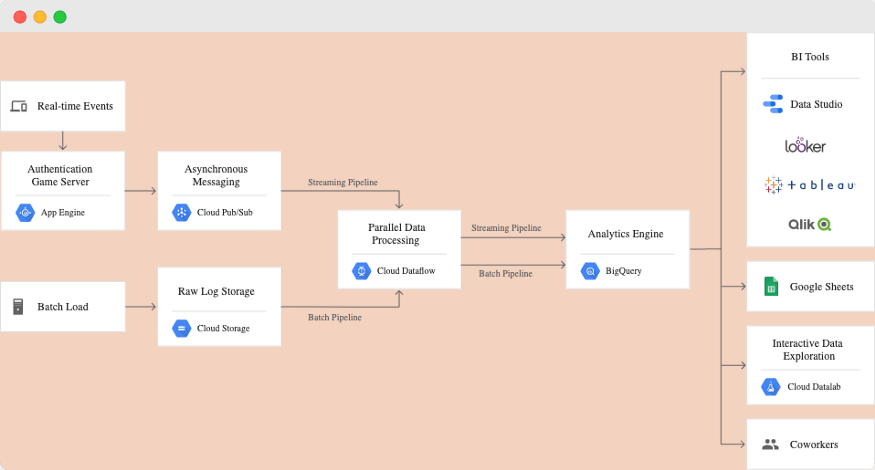
[Example of integration BigQuery into a data processing solution with different front-end integrations]
BigQuery is a data warehouse. BigQuery allows us to store and query massive datasets of up to hundreds of Petabytes. BigQuery is very familiar to relational databases by their structure. It has a table structure, uses SQL, supports batch and streaming writing into the database, and is integrated with all Google Cloud Platform services, including Dataflow, Apache Spark, Apache Hadoop, etc. It’s best for use in interactive queuing and offline analytics.
Benefits
- Huge capacity, up to hundreds of Petabytes
- SQL
- Batch and streaming writing
- Support complex queries
- Built-in ML
- Serverless
- Shared datasets — you can share datasets between different projects
- Global locations
- All popular data processing tools have interfaces to BigQuery
Limitations
- It doesn’t support transactions, but those who need transitions in the OLAP solution
- The maximum size of the row is 10Mb
Pricing strategy
You pay separately for stored information(for each Gb) and executed queries.
You can choose one of two payment models concerning executed queries, either paying for each processed Terabyte or a stable monthly cost depending on your preferences.
Analogs & alternatives
- Amazon Redshift
- Azure Cosmos DB
Cloud BigTable
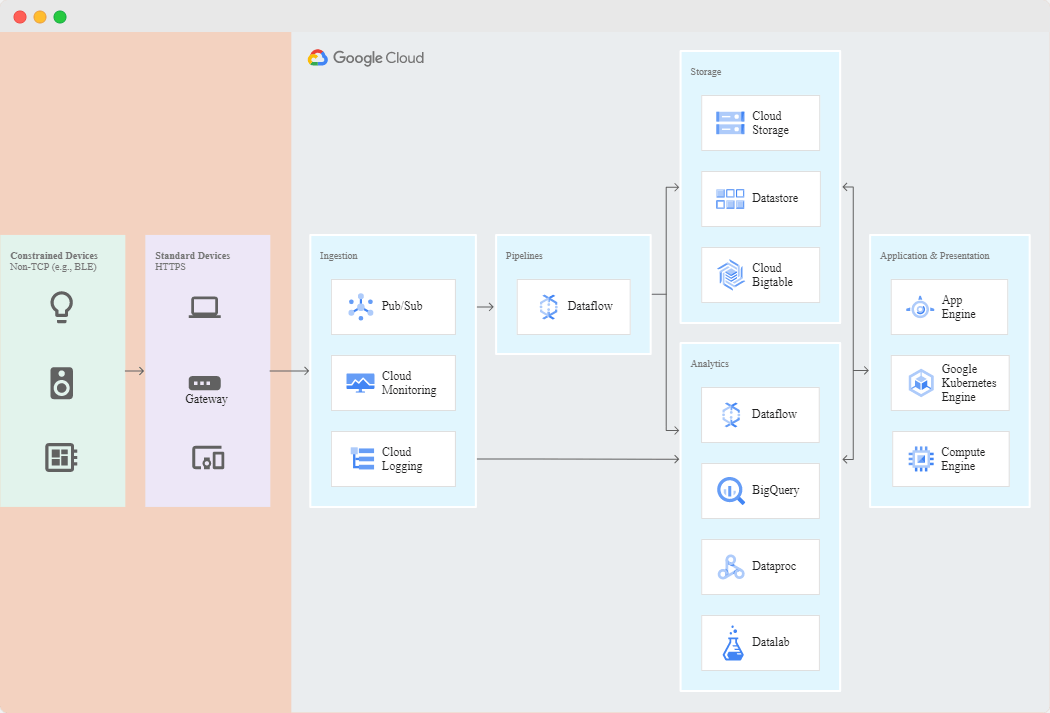
Google Cloud BigTable is Google’s NoSQL Big Data database service. The same database powers many core Google services, including Search, Analytics, Maps, and Gmail. Bigtable is designed to handle massive workloads at consistent low latency and high throughput, so it’s an excellent choice for operational and analytical applications, including IoT, user analytics, and financial data analysis.
Cloud Bigtable is based on Apache HBase. This database has an enormous capacity and is suggested for use more than Terabyte data. One example, BigTable is the best for time-series data and IoT data.
Benefits
- Has good performance on 1Tb or more data
- Cluster resizing without downtime
- Incredible scalability
- Support API of Apache HBase
Limitations
- Has bad performance on less than 300 Gb data
- It doesn’t suit real-time
- It doesn’t support ACID operations
- The maximum size of a single value is 100 Mb
- The maximum size of all values in a row is 256 Mb
- The maximum size of the hard disk is 8 Tb per node
- A minimum of three nodes in the cluster
Pricing Strategy
BigTable is very expensive. You pay for nodes (minimum $0.65 per hour per node) and storage capacity(minimum 26$ per Terabyte per month)
Analogs & alternatives
- Custom deployed Apache HBase
Cloud Storage
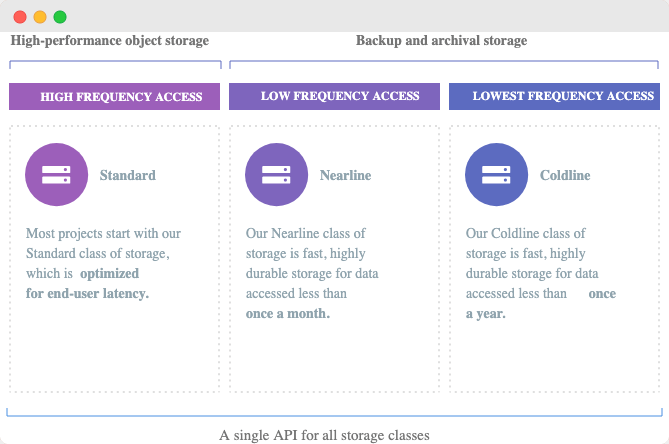
GCS is blob storage for files. You can store any amount of any size files there.
Benefits
- Good API for all popular programming languages and operating systems
- Immutable files
- Versions of files
- Suitable for any size files
- Suitable for any amount of files
- Etc
Pricing Strategy
GCS has a couple of pricing plans. In a standard plan, you pay for 1Gb of saved data.
Analogs & alternatives
- Amazon S3
- Azure Blob Storage
How to make your IT project secured?
Download Project Security ChecklistOther Google Cloud Services
There are a few more services that I should mention.
Google Cloud Compute Engine provides virtual machines with any performance capacity.
Google CloudSQL is a cloud-native solution to host MySQL and PostgreSQL databases. Has built-in vertical and horizontal scaling, firewall, encrypting, backups, and other benefits of using Cloud solutions. Has a terabyte capacity. Supports complex queries and transactions
Google Cloud Spanner is a fully managed, scalable, relational database service. Supports SQL queries, auto replication, transactions. It has a one-petabyte capacity and suits best for large-scale database applications which store more than a couple of terabytes of data.
Google StackDriver monitors Google services and infrastructure, and your application is hosted in a Google Cloud Platform.
Cloud Datalab is a way to visualize and explore your data. This service provides a cloud-native way to host Python Jupyter notebooks.
Google Cloud AutoML and Google AI Platform allow training and hosting of high-quality custom machine learning models with minimal effort.
Conclusion
Now you are familiar with the primary data services that Google Cloud Platform provides. This knowledge can help you to build a good data solution. But, of course, Clouds are not a silver bullet, and in case you use Clouds in the wrong way, it can significantly affect your monthly infrastructure billing.
Thus, carefully build your proposal’s architecture and choose the necessary services for your needs to reach your needed business goals. Explore all benefits and limitations for each particular case. Care about costs. And, of course, remember about the scalability, reliability, and maintainability of your solution.
Useful links: