The role of AI and machine learning in digital biology
Digital biology, also known as biotechnology and digital biotech, gives bioengineers, medication producers, agricultural companies, and industrial businesses excellent opportunities. Biotechnicians can turn biomaterials – living systems and organisms – into a digital data format, organize it, discover hidden patterns, and store it in databases.
Why does it matter?
Such an approach streamlines the research, development, and test stages of biology projects that previously took bio technicians months or years. Moreover, medical specialists apply digital biology to diagnose health conditions, such as cancer and sepsis, within several hours and suggest the most appropriate treatment based on patient samples.
Digital biology took a leap in development by applying Artificial intelligence and machine learning algorithms that automate biological data analysis and research. Thus, bioengineers generate more data in shorter terms, compared with the analog study methods they used previously.
In this article, you’ll find the current state of digital biology and the fields it serves. You’ll also read about biotechnology areas that benefit the most from other intelligent technologies, such as AI, machine learning, and cloud computing.
The current state of the Digital Biology market
Digital biology is a cross-disciplinary field that combines both biological and technological components. It includes exploring and analyzing living organisms with new intelligent tools.
Recognizing the considerable potential of biotechnology, governmental organizations, such as the National Institute of Biomedical Imaging & Bioengineering in developed nations and the National Center for Biotechnology Information, increased their investments into the research and development activities in biotechnology fields.
The market research from Global Market Insights (GMI), a global market research and management consulting company, says an increased interest from governmental organizations is expected to make biotechnology the largest and the fastest-growing market, projected to reach $729 billion by 2025, compared with $417 billion in 2018.
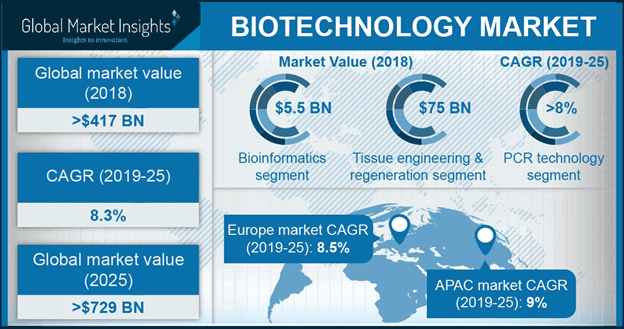
The research also includes a forecast of revenue increase for the following technology segments:
- Fermentation
- Tissue engineering and regeneration
- PCR technology
- Nanobiotechnology
- Chromatography
- DNA sequencing
- Cell-based assay
And others.
In particular, the fermentation segment is the most prominent sub-niche of biotechnology that received an 11% revenue share of the whole biotechnology market in 2018.
The report predicts substantial progress for fermentation technology during the next few years. Fermentation is a process that changes organic substrates on the chemical level by enzyme action and micro-organisms.
Such growth of fermentation technology is explained by excessive use in the food and beverage industry. The food and beverage industry’s key business players will increase investments in biotechnology to improve the research and development activities to produce more fermented products.
The expected growth of biotechnology opens new opportunities for biotech startups, well-established companies, and research institutions. Another reason for biotechnology’s rise is various applications in medication, agriculture, and other industries.
Biotechnology Application Outlook
Digital biology, or biotechnology, includes several categories of applications. Biological technicians and other scientists apply digital biotechnology for solving scientific problems with living organisms across various industries – from healthcare and agricultural to industrial processing and bioinformatics.
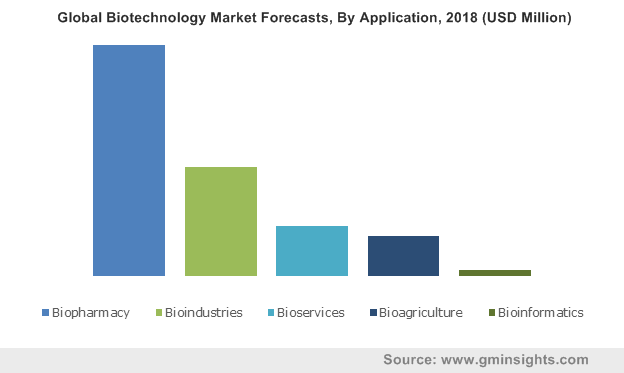
Let’s see how each category benefits from artificial intelligence and machine learning.
Health
In medical biotechnology, scientists receive information from living cells to get a clearer picture of human health, thus producing the most appropriate drugs and antibiotics.
Bio technicians dig into the smallest details to achieve these goals – study DNA and manipulate cells to predict beneficial and vital characteristics.
The most useful tech solutions used in medical biotechnology are Artificial Intelligence and machine learning that enable scientists to improve their drug discovery process by reaching small molecules and their target structures they need to treat.
Machine learning algorithms also perform great for patient testing and diagnostics. The algorithm can detect damaged tissues and other abnormalities via medical images, patient samples, and even sounds. For example, intelligent algorithms can detect cancer cells in X-rays, sepsis via DNA sequencing, and define whether the patient has COVID after hearing one’s cough.
In this way, doctors provide more timely and accurate treatment for better outcomes.
Moreover, artificial intelligence and machine learning are used in electronic health record (EHR) systems and clinical decision support systems to help doctors suggest a patient’s personalized medical treatment and accurate medication management.
Food and agriculture
Agricultural biologists apply biotechnology to increase crop yields, genetically modified plants, and identity infected crops before the harvest. For these purposes, scientists use DNA sequencing devices and databases with DNA samples of already sequenced genes. Once new DNA samples are sequenced, scientists can change their structure, learn more about the plant origins and potential issues typical for one or other plant.
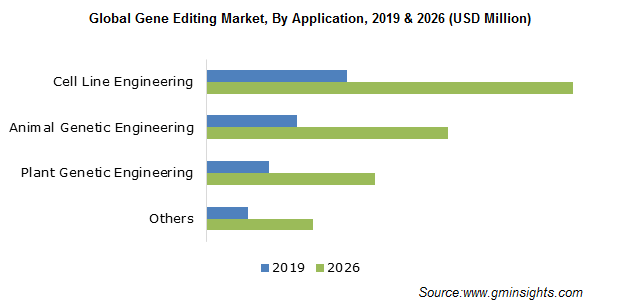
[Increasing application of cell line engineering will drive the overall market expansion]
Food and agriculture biotechnology companies apply AI-algorithms to harvest crops, watch crop health, and find AI-powered tools more effective than humans.
Such an application requires food and agriculture businesses to integrate autonomous robots or drones, computer vision algorithms, and deep learning technologies. While drones and robots carry cameras, algorithms analyze crop pictures they receive, compare data captured with crop images in their database, and define whether crops and soil are healthy or not.
Industrial processing
Industrial biotechnology includes research on biopolymer substitutes, inventions of vehicle parts, alternative fuels, new chemicals, and the process of production. In this area, intelligent technologies and the Internet of Things (IoT) devices help industrial producers analyze their machinery to predict outages, optimize equipment, and even reduce human worker numbers with automated warehouse management.
One example is Ocado Technology, an online grocery retailer that automated its warehouse with 3500 robots to process 220,000 online orders a week for grocery delivery.
To learn more about AI and machine learning applications in industrial processing and supply chain, check out our previous article about top AI applications in supply chain optimization.
Bioinformatics
Bioinformatics is a subdiscipline of digital biology that combines biology and computer science to acquire, store, analyze, and disseminate biological data, such as DNA and amino acid sequences. Scientists understand biological information using mathematics, data science, and different digital biology tools by organizing it in large biological pools.
Bioinformatics also receive benefits from AI and machine learning. Artificial intelligence and machine learning help biologists sequence DNA from the massive data crunch, classify proteins, protein catalytic roles, and their biological functions. Leveraging intelligent technologies, scientists can automate gene expressions and gene annotation and identify the location of genes required for computer-aided medication design.
In digital biology, biotechnologists base their research on digital data, generated from life samples or DNA sequencing devices and stored in a thousand databases, both private and public.
So we can conclude that the growing biotechnology industry will heavily rely on AI-algorithms, machine learning, and data analytics. But the development of biotechnology across all segments depends on biotechnology researchers’ ability to master their skills for the useful contribution of their findings and researcher results. Not only AI makes biotech engineers more efficient, but also for a bunch of other reasons.
Let’s check them out.
Top 3 advantages of using AI in the biotechnology industry
PwC’s Global Artificial Intelligence Study: Exploiting the AI Revolution says AI will contribute to the global output of $15.7 trillion by 2030. By this time, 44% of pharma and life sciences experts expect to adopt Artificial Intelligence in their laboratories and R & D centers and replace analog tests.
But why do scientists prefer digital biology to the old-but-gold analog approach?
Development and research projects often require scientists to deal with numerous amounts of data and large sample sizes, such as genome sequencing. In such cases, biological test digitalization allows researchers to produce more data than analog study methods. And by applying digital biology, scientists can receive real-time insights into biological functions which have taken them days and weeks when using an analog approach.
The adoption of AI and machine learning by biology specialists make the digital biology approach even more useful. And here is how:
Crucial predictions
Artificial intelligence and machine learning algorithms help bio technicians make more precise predictions than standard approaches used for decades. Successfully applied in supply chain and logistics, predictive analytics drastically reduce the time biotech companies spend to launch new products to market.
To make data-based decisions and forecast outcomes, data scientists train algorithm models with historical databases. Then, such algorithms are effectively used for pattern recognition, despite the data type.
As Nature online resource highlighted, intelligent algorithms’ ability to analyze large amounts of data in datasets helps drug-producing companies make new pharmaceuticals quicker and more effective. Soon, medication specialists will provide more personalized treatments, based on the disease’s cause, hidden deeply in biological structures. In this way, pharmaceutical companies can reduce the medicine development process from the $2.6 billion price tag and decrease the 90% failure rate of new medication created.
In her article, Melanie Matheu, Ph.D. and founder at Prellis Biologics, Inc. the human tissue engineering company predicts the new generation of therapeutics entering drug pipelines empowered by AI screening for selecting targets will reduce clinical trial failure rates for small molecules by 86%.
Effective decision-making
Clinical trials used to be manual and a very time-consuming process – they included inviting participants to the clinic during the in-person visit, recording their symptoms, prescribing them treatments, and analyzing side effects. Moreover, to get the right sample size, medication companies heavily invested in marketing resources for recurring right patients and treating rare conditions.
Now, intelligent algorithms and cloud technologies digitized clinical trials and enabled biotech organizations to test medication on more patients within less time.
One example is Invitae, a medical genetic company. In November 2019, the company launched a trial in collaboration with Apple Watch to bring together biometric data from wearables and genetic tests and determine genes that cause cardiovascular disease. In this way, the company made the trial available to many people and excluded Apple Watch users who didn’t meet the trial criteria.
Biotech companies make clinical trials even more effective by leveraging machine learning algorithms that analyze data from current trials and use it for forecasting treatment effectiveness in the future, down to a molecular level. ML also helps scientists revise information from previous tests to find gaps and new applications for existing medications.
Cost-effectiveness
Modern devices, cloud databases, data analytics pipelines, and machine learning algorithms reduced the cost of genome sequencing from $2.7 billion for the Human Genome Project to less than $300 by now. It is expected to cost even less – $100 in the future. Bioengineers receive more extensive screening of trial participants and targeting of interventions. They also see the future in personalized treatment plans and targeted therapies that provide therapies at genetic and molecular levels of patient genes.
The main area for targeted therapy is cancer treatment – the treatment of blood cancer such as leukemia, where a treatment called CAR T-cell therapy, according to the National Cancer Institute, the immune system will “attack tumors,” so we’ll soon witness more cancer survivors.
Biotech organizations also use cloud computing to host and run computations and no longer need to buy expensive computer hardware for their research. This fact is a substantial benefit for early-stage startups with limited funding to enter the market with their research and medications. Cloud computing is also handy for established medical corporations, allocating resources for new projects cheaper and more manageable.
What is the future of AI and machine learning in the biotechnology industry?
Biotechnology is an innovative industry that effectively solves scientific problems with living organisms. But new issues continuously arise and require biotechnologists applying modern methods to be solved.
Thus, to remain relevant, biotech specialists must make room for improvements. Fortunately, there are many solutions they can apply – AI, data analytics, deep learning, and others we’ve already listed in this article.
Thus, AI, machine learning, and robotics play critical roles in pushing the boundaries of possibilities in medical, industrial, or agricultural biotechnologies, and will remain relevant for subsequent decades.
The APP Solutions has experience developing and integrating AI functionality into biotech projects. You can learn more about our expertise in creating a real-time DNA sequence analysis application during our partnership with the Google Cloud Platform and the Queensland University of Technology. Don’t hesitate to contact us if you need experts to advise and develop intelligent software for your biotech project.
What our clients say
Related reading: