Machine learning in mobile applications: best examples
- Reasons to build a machine learning app
- Types of Machine Learning algorithms for Android and iOS apps
- Industry-specific application areas of machine learning apps
- Top Machine Learning Mobile Application Examples
- Snapchat
- Tinder
- Spotify
- Yelp
- eBay
- Machine learning in mobile apps: Closing Thoughts
Have you ever wondered how Facebook automatically tags you on your friend’s photo? Or why Spotify has such great songs in “Discover Weekly”? The answer is because these companies use machine learning in mobile applications to provoke “WOW” reactions from users.
If you are considering integrating machine learning into your app, you are in the right place. In this article, we gather the main types of machine learning algorithms and share ideas on how to use machine learning for an industry-specific mobile app. We will also pick the best examples of machine learning practical applications and explain how they work.
But first, let’s find out why you should integrate machine learning into your mobile application.
Reasons to build a machine learning app
What benefits you can expect from implementing machine learning
- After ML integration, 76% of businesses recorded higher sales
- ML technology predicts better user behavior, optimizes processes, lead up-sell, and cross-sell
- 50% of enterprises are applying machine learning to refine marketing issues
- ML has helped several European banks to increase new product sales by 10%
Now, let’s dig deeper into technologies that power Machine Learning algorithms.
Emerging Trends in Mobile ML to Watch in 2025
With a mobile ML product, it has become crucial to adapt to market changes and introduce new techniques and trends. The latter helps companies fight competition effectively, prove their worth, and secure their position in the niche. Here are three emerging trends to watch in 2025 that help to stay on the beaten track.
On-device ML. Improving a core with new features and frameworks like Core ML or TensorFlow Lite is not only trendy but necessary, as they reduce cloud dependency and enable crucial improvements for better data processing and a privacy-focused environment.
Continuous learning. Machine learning is not new, but it is still the talk of the town as more niches start to recognize its enormous potential and introduce cutting-edge approaches for decentralized learning in their applications.
Multimodal AI. To provide users with a truly immersive experience, leading companies have started to leverage multimodal AI to make the most of different types of data, such as text, image, audio, video, and sensor.
Types of Machine Learning algorithms for Android and iOS apps
Machine Learning (ML) is a technology of automated data processing and decision-making algorithms. Such algorithms improve their operation according to the results of their work. This is a form of “learning on the go.”
The more qualified data ML apps have, the more accurate the ML algorithm becomes.
To build a model that uncovers connections, Machine Learning uses the following three algorithms:
- Supervised learning, when an algorithm learns from example data and associated target responses. This data might include numeric values or string labels such as classes or tags. Later, when posed with new examples, ML can predict the correct response.
- Unsupervised learning. ML learns from examples without any associated answers. Thus, the algorithm determines the data patterns on its own.
- Reinforcement Learning. Developers train ML algorithms to make specific decisions from the environment. In this way, the machine captures the best possible knowledge to make accurate decisions.
Related reading:
MULTILAYER PERCEPTRON NETWORKS APPLICATIONS & EXAMPLES OF BUSINESS USAGE
DO YOU NEED A MOBILE E-COMMERCE APP FOR YOUR STORE?
Industry-specific application areas of machine learning apps
There are numerous applications of machine learning. Thus, you can choose several machine learning use cases for a mobile app from any industry. Here are some use cases for machine learning in industry-specific mobile applications.
-
AI-powered financial assistant
Let’s see what machine learning is used in finance. You can use such mobile applications, powered to receive insights into your personal finances. In most cases, such apps are developed by banks to provide clients with additional value. By leveraging machine learning algorithms, the app analyzes your transaction history and comes up with expenditure predictions, track spending habits, and gives financial advice. An example of such an app is Erica, a mobile voice assistant, developed by the Bank Of America. Currency, over Erica’s financial assistant, provides more personalized and convenient banking to 25 million mobile app customers.
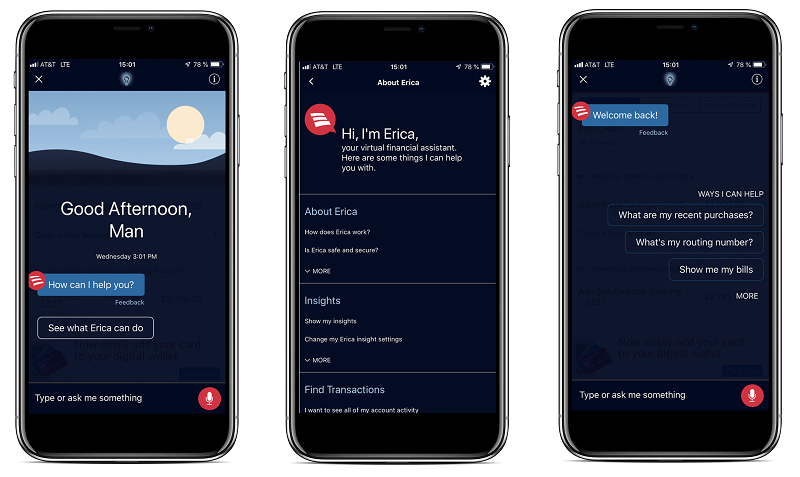
-
Fitness mobile apps with ML
Such workout apps, powered with machine learning capabilities, analyze data gathered from wearables, smartwatches, and fitness trackers. Then, depending on one’s goal, the user receives personalized lifestyle advice. The algorithm also analyzes the user’s current fitness levels and eating habits to provide customized fitness plans. Aaptiv Coach is one of the most prominent fitness apps with machine learning. The app gives users an order of workouts, including custom workouts from Aaptiv, and measures the user’s progress.
-
Healthcare mobile applications with ML
Numerous condition-based mobile applications help users to keep track of heart illnesses, diabetes, epilepsy, and migraines. Thanks to machine learning algorithms, such apps analyze user input, predict the possibility of one or other conditions, and notify the doctor about the patient’s current condition for streamlined treatment.
-
Transportation mobile applications
Logistics mobile apps, such as Uber for trucking or fleet management, need to provide the driver with up-to-date information about traffic conditions. Then, based on current situations, such apps optimize roads to avoid traffic jams, deliver cargo on time, and avoid extra fuel consumption. To receive such traffic information beforehand, developers integrate traffic prediction software with machine learning algorithms into road optimization mobile apps. The algorithm analyses historical data about traffic conditions and predicts the traffic patterns for a particular day and time. To learn more about other applications of machine learning in transportation, read the article How AI is changing the logistics industry.
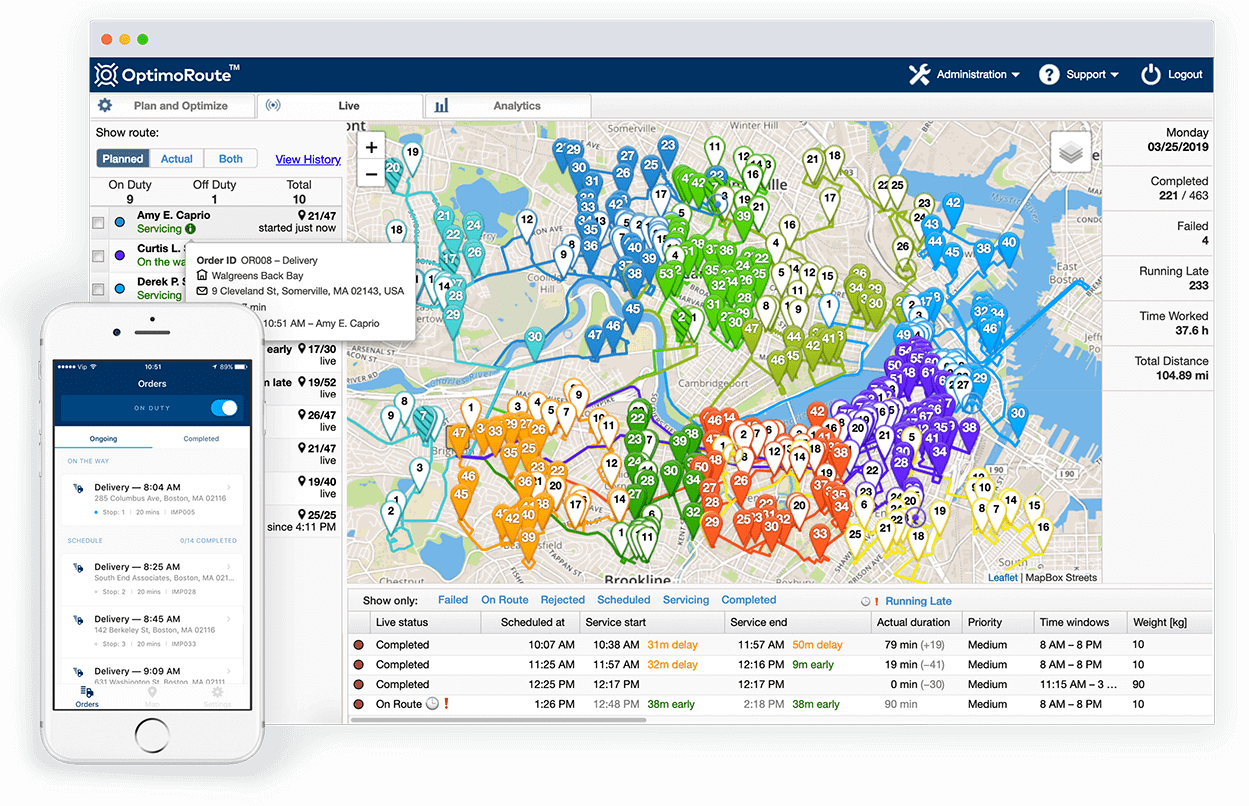
[Optimoroute, ML route optimization mobile app, source]
-
E-commerce
Online retail mobile apps can use machine learning algorithms in several ways. For example, such algorithms are handy to provide the buyer with more relevant product recommendations based on purchase history, identify fraud with credit cards, and visual search. If you are looking for more applications of machine learning in mobile e-commerce apps, don’t forget to check the article How online clothing retailers can leverage AI for online shopping.
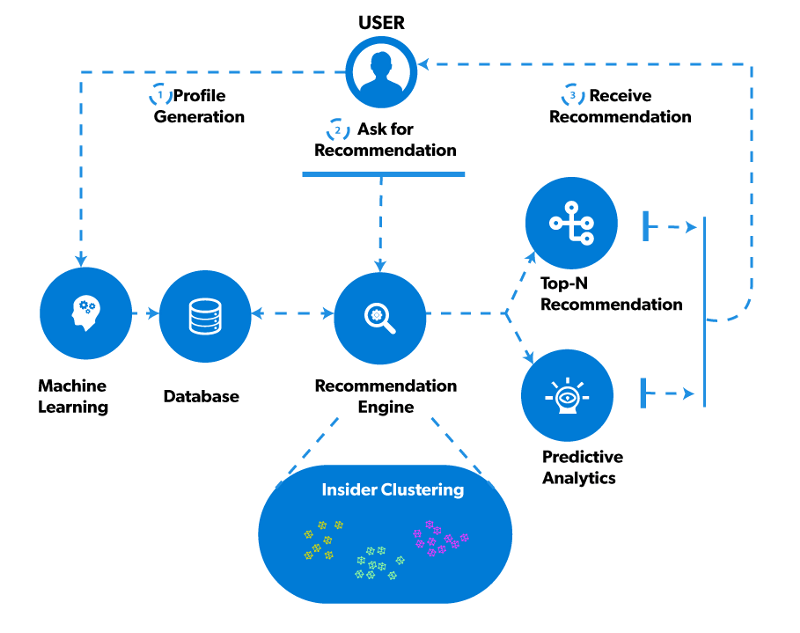
[Source]
Related reading:
HOW E-COMMERCE USES MACHINE LEARNING APPLICATIONS
DO YOU NEED A MOBILE E-COMMERCE APP FOR YOUR STORE?
Top Machine Learning Mobile Application Examples
Advanced algorithms transform the way users interact with their devices while bringing unique machine learning mobile app ideas. The list below includes the best apps that use machine learning.
Snapchat
This application uses machine supervised learning algorithms for computer vision. Looksery, a Ukrainian startup, developed the algorithm for computer vision. Soon, Snapchat acquired this company for $150 million. Now, the mobile machine learning algorithm finds faces in photos to add fun elements like glasses, hats, dog ears, and more. In this article, we have given a more detailed explanation of how ML Snapchat filters work.
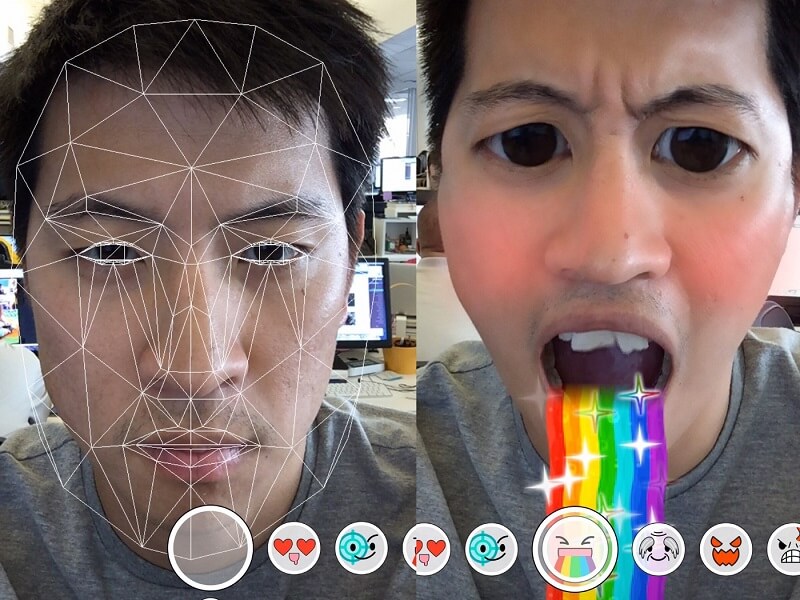
[Source]
Tinder
The app uses an algorithm with reinforcement learning for the ‘Smart Photos’ feature, increasing the user’s chances of finding the perfect match. The app shows user photos to other users in random order. Then, Machine Learning analyzes how many right or left swipes each image receives. In this way, Tinder learns which photos are more attractive to other users. The algorithm reorders the user photos to put popular images first.
Download Free E-book with DevOps Checklist
Download NowRelated reading:
HOW TO DEVELOP A DATING APP LIKE TINDER
Spotify
Spotify leverages three types of machine learning algorithms to provide users with personalized music recommendations in the ‘Discover Weekly Section and increases engagement.
The first type of algorithm is Collaborative Filtering. This algorithm provides users with personalized recommendations by comparing multiple user-created playlists with songs users have listened to. Then, the algorithm combs those playlists to look at other songs from users’ playlists and recommends them.
The second ml algorithm with Natural Language Processing ‘reads’ song lyrics, blog posts and discussions about specific musicians, and news articles about songs or artists on the internet. Then, based on this information, the algorithm categorizes into “cultural vectors” and “top terms” and suggests music with similar meaning.
The third algorithm is the Audio model. Machine learning tools analyze data from raw audio tracks, categorize songs, and suggest other songs with similar music, popular among other users.
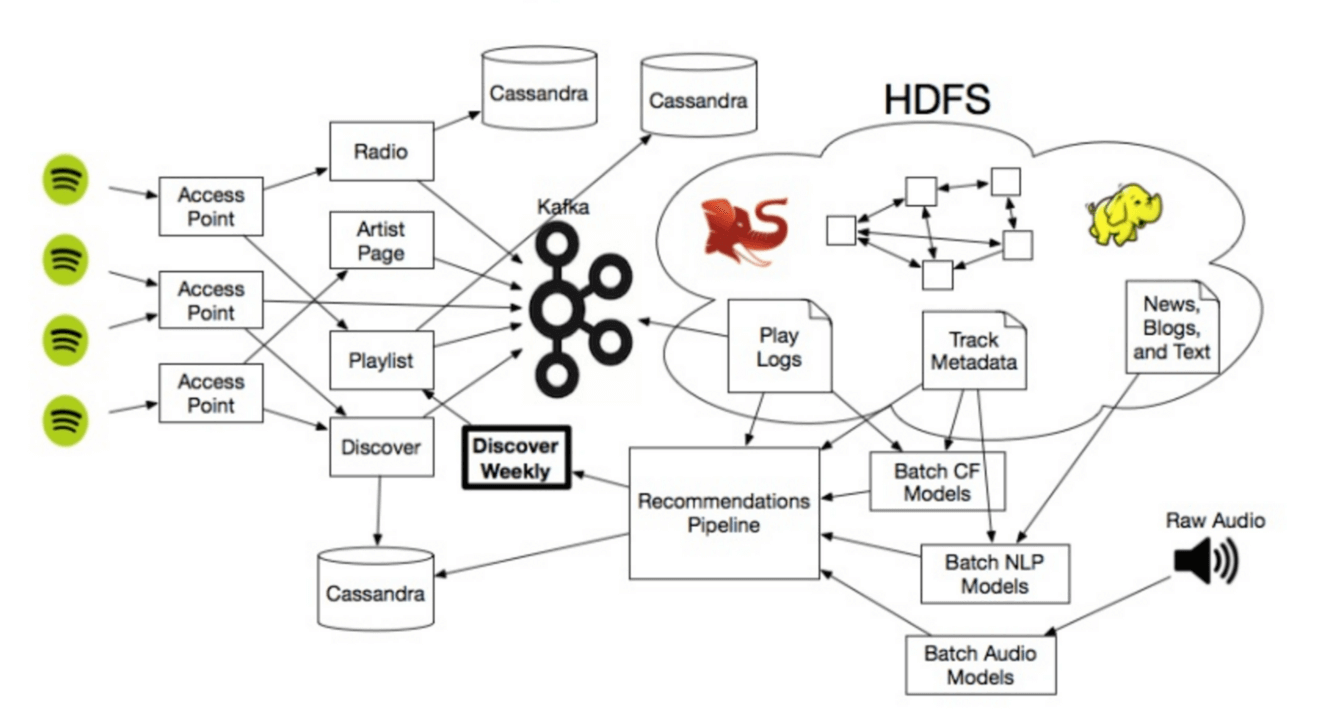
[Discover Weekly data flow, source]
Yelp
This app uses supervised Machine Learning to enhance user experience with “Recommended for You” collections. The ML algorithm skims the reviews for each restaurant listed. Then, ML determines the most popular dishes on how many times the meal was mentioned. Besides this, Yelp uses ML to accumulate, classify, and label user-submitted photos of dishes with different attributes. Such attributes include “ambiance is classy” or “good with kids” with 83% accuracy.
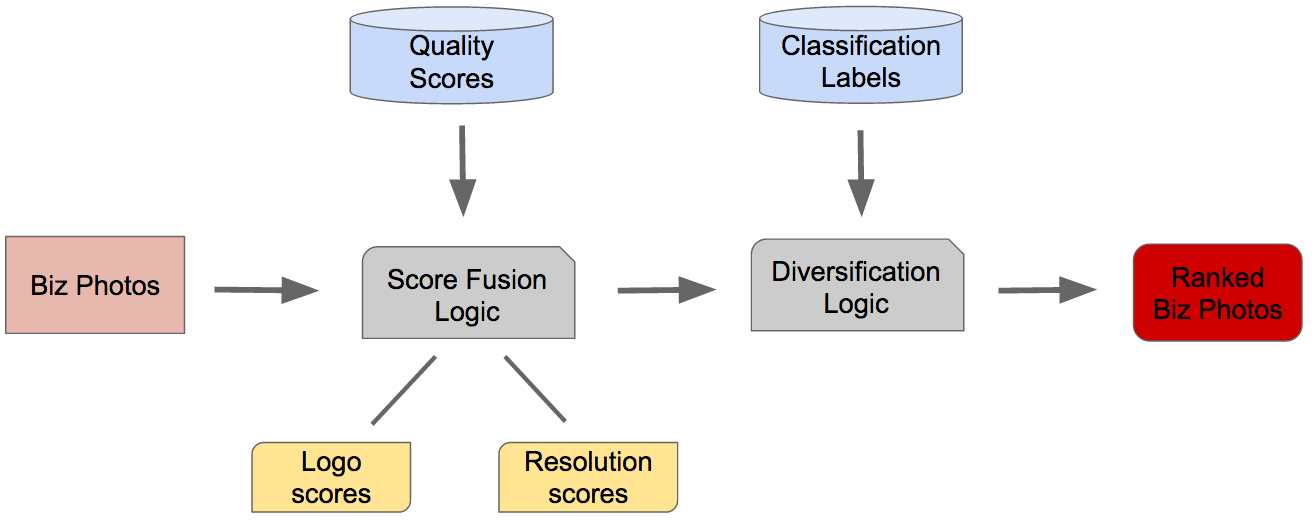
[Yelp business photo ranking pipeline, source]
Facebook applies machine learning algorithms in several ways. For instance, the network suggests your new friends in the “People You May Know” section after the ml algorithm analyzes your profile and interests, your current friends, and their friends. The algorithm also gathers other factors and comes up with suggestions on people you may know. Other aspects where Facebook uses machine learning are Newsfeed, targeted ads, and facial recognition.
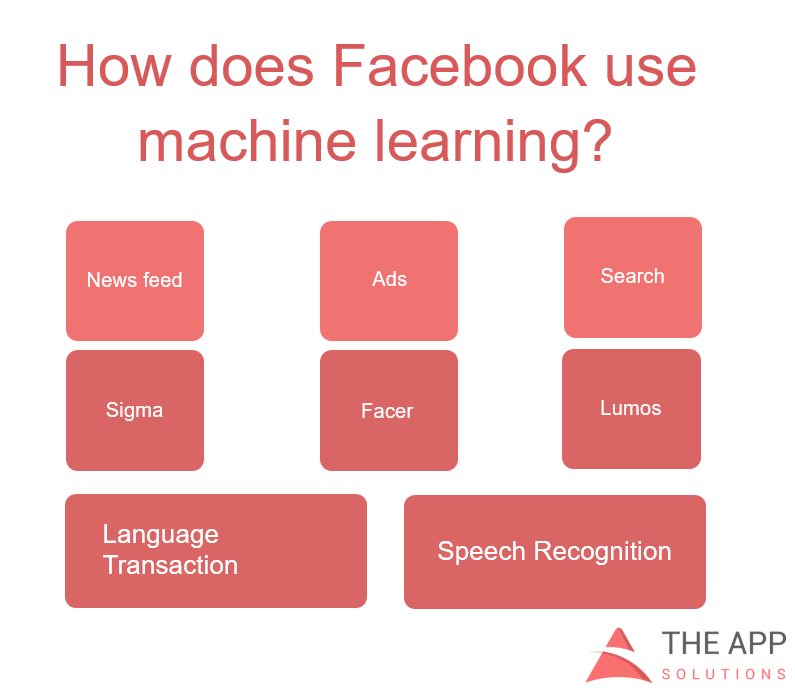
Want to Learn More About The APP Solutions Approaches?
Download Free EbookeBay
This online store applies a Reinforcement Machine Learning algorithm for the product recommender Chabot, called ShopBot. The Machine Learning algorithm helps ShopBot to understand what users are looking for. Chatbot then processes their text messages and images and finds the best match. eBay chat-bot has been praised for its excellent contextual understanding, as well as its use of friendly language.
As a result, ShopBot users are three times more likely to ask questions about specific products than those browsing eBay’s inventory.
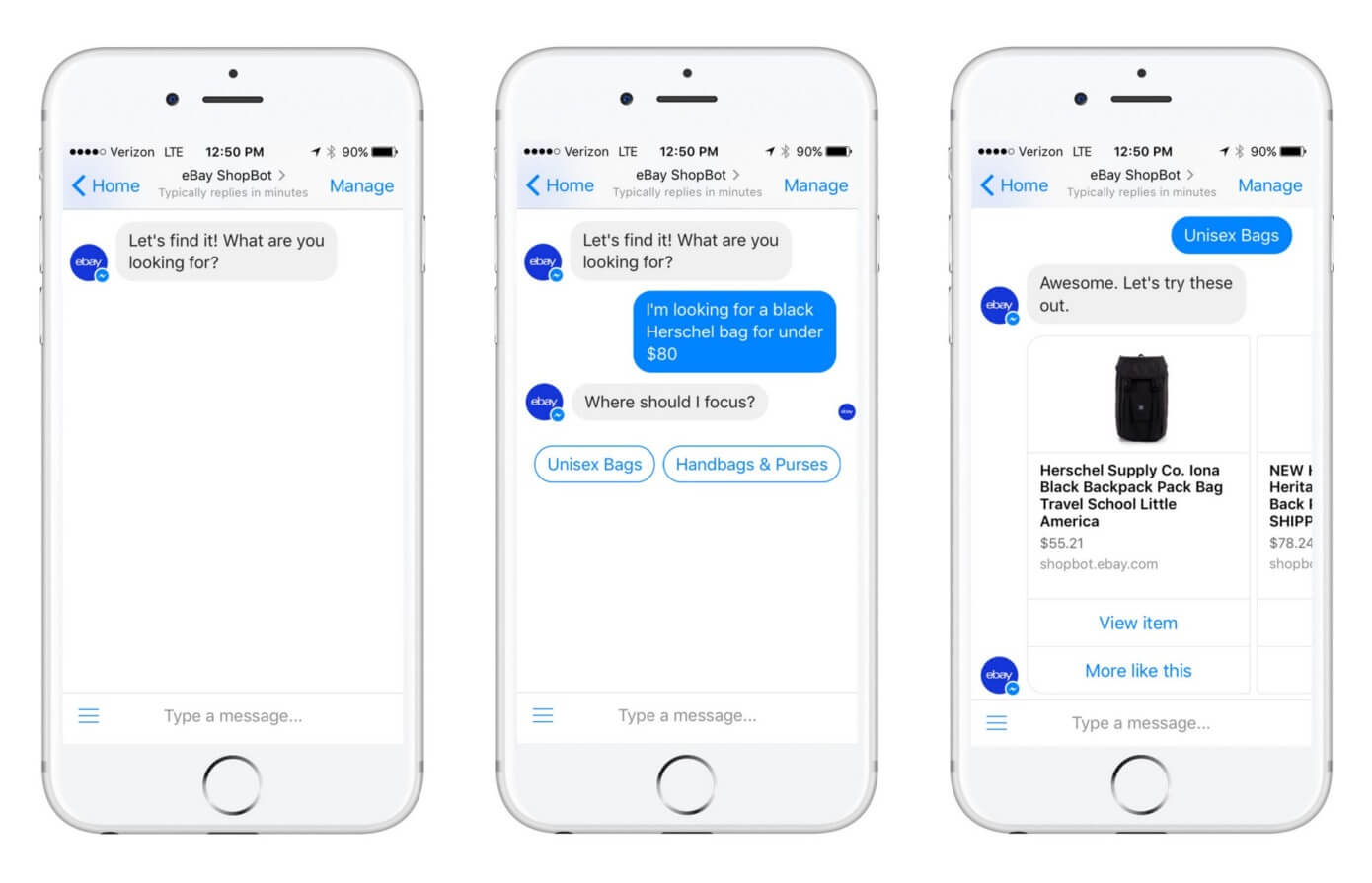
[Source]
Machine learning in mobile apps: Closing Thoughts
ML algorithms improve customer experience, maintain customer loyalty, increase engagement, and so on. This technology suits any mobile business app that needs predictions and has a large enough data set.
There are many application areas of machine learning in many industries, such as banking, healthcare, transportation, and e-commerce. Depending on your business needs, you can leverage one of the ML algorithms described in this article. The last remaining thing is to hire an experienced team for machine learning app development.
HOW TO OUTSOURCE APP DEVELOPMENT