4 Types of Machine Learning Algorithms
Machine Learning came a long way from a science fiction fancy to a reliable and diverse business tool that amplifies multiple elements of the business operation.
Its influence on business performance may be so significant that the implementation of machine learning algorithms is required to maintain competitiveness in many fields and industries.
The implementation of machine learning in business operations is a strategic step and requires a lot of resources. Therefore, it’s important to understand what do you want the ML to do for your particular business and what kind of perks different types of ML algorithms bring to the table.
In this article, we’ll cover the major types of machine learning algorithms, explain the purpose of each of them, and see what the benefits are.
Types of Machine Learning Algorithms
Algorithms include supervised and unsupervised learning systems as well as Reinforcement and Semi-supervised machine learning technology.
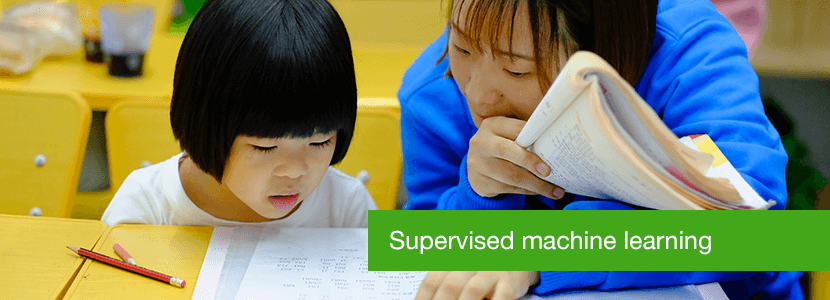
Supervised Learning Algorithms
Supervised Learning Algorithms are the ones that involve direct supervision (cue the title) of the operation. In this case, the developer labels sample data corpus and set strict boundaries upon which the algorithm operates.
It is a spoonfed version of machine learning:
- you select what kind of information output (samples) to “feed” the algorithm;
- what kind of results it is desired (for example “yes/no” or “true/false”).
From the machine’s point of view, this process becomes more or less a “connect the dots” routine.
The primary purpose of supervised learning is to scale the scope of input data and to make predictions of unavailable, future or unseen data based on labeled sample data.
DOES YOUR BUSINESS REALLY NEED AN ENTERPRISE ARTIFICIAL INTELLIGENCE
Supervised machine learning includes two major processes: classification and regression.
- Classification is the process where incoming data is labeled based on past data samples and manually trains the algorithm to recognize certain types of objects and categorize them accordingly. The system has to know how to differentiate types of information, perform an optical character, image, or binary recognition (whether a particular bit of data is compliant or non-compliant to specific requirements in a manner of “yes” or “no”).
- Regression is the process of identifying patterns and calculating the predictions of continuous outcomes. The system has to understand the numbers, their values, grouping (for example, heights and widths), etc.
The most widely used supervised algorithms are:
- Linear Regression
- Logistical Regression
- Random Forest
- Gradient Boosted Trees
- Support Vector Machines (SVM)
- Neural Networks
- Decision Trees
- Naive Bayes
- Nearest Neighbor
Supervised Learning Algorithms Use Cases
The most common fields of use for supervised learning algorithm is price prediction and trend forecasting in sales, retail commerce, and stock trading. In both cases, an algorithm uses incoming data to assess the possibility and calculate possible outcomes.
The best examples are Sales enablement platforms like Seismic and Highspot use this kind of an algorithm to present various possible scenarios for consideration.
Business cases for supervised learning method include ad tech operations as part of the ad content delivery sequence. The role of the supervised learning system there is to assess possible prices of ad spaces and its value during the real-time bidding process and also keep the budget spending under specific limitations (for example, the price range of a single buy and overall budget for a certain period).
WHAT IS THE DIFFERENCE BETWEEN WEB 2.0 VS. WEB 3.0?
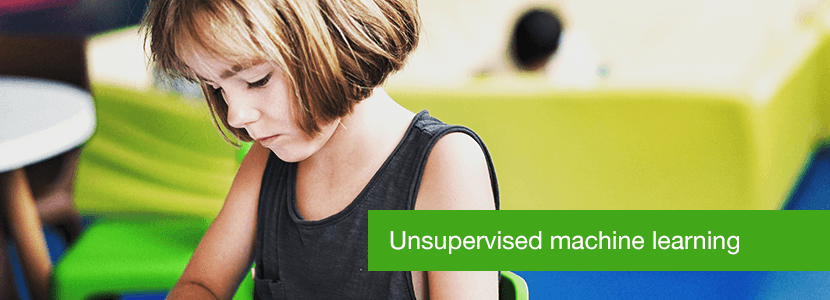
Unsupervised Learning Algorithms
Unsupervised Learning is one that does not involve direct control of the developer. If the main point of supervised machine learning is that you know the results and need to sort out the new data, then in the case of unsupervised learning algorithm the desired results are unknown and yet to be defined.
Another big difference between the two is that supervised learning uses labeled data exclusively, while unsupervised learning feeds on unlabeled data.
The unsupervised machine learning algorithm is used for:
- exploring the structure of the information;
- extracting valuable insights;
- detecting patterns;
- implementing this into its operation to increase efficiency.
In other words, unsupervised learning techniques describe information by sifting through it and making sense of it.
Unsupervised learning algorithms apply the following techniques to describe the data:
- Clustering: it is an exploration of data used to segment it into meaningful groups (i.e., clusters) based on their internal patterns without prior knowledge of group credentials. The credentials are defined by the similarity of individual data objects and also aspects of their dissimilarity from the rest (which can also be used to detect anomalies).
- Dimensionality reduction: there is a lot of noise in the incoming data. Machine learning algorithms use dimensionality reduction to remove this noise while distilling the relevant information.
The most widely used algorithms are:
- k-means clustering
- t-SNE (t-Distributed Stochastic Neighbor Embedding)
- PCA (Principal Component Analysis)
- Association rule
DATA MINING VS. PREDICTIVE ANALYTICS: KNOW THE DIFFERENCE
Use Cases of Unsupervised Learning Algorithms
Digital marketing and ad tech are the fields where unsupervised learning is used to its maximum effect. In addition to that, this algorithm is often applied to explore customer information and adjust the service accordingly.
The thing is – there are a lot of so-called “known unknowns” in the incoming data. The very effectiveness of the business operation depends on the ability to make sense of unlabeled data and extract relevant insights out of it.
AI /ML FOR ELECTRONIC HEALTH RECORDS
Unsupervised algorithms equip modern data management. At the moment, Lotame and Salesforce are among the most cutting-edge data management platforms that implement this machine learning system.
As such, unsupervised learning can be used to identify target audience groups based on certain credentials (it can be behavioral data, elements of personal data, specific software setting or else). This algorithm can be used to develop more efficient targeting of ad content and also for identifying patterns in the campaign performance.
THE ROLE OF AI AND MACHINE LEARNING IN DIGITAL BIOLOGY
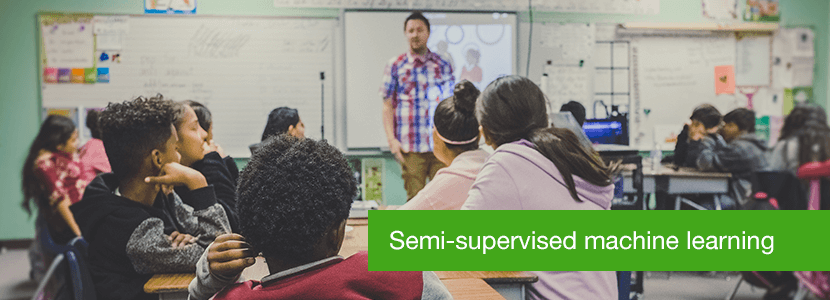
Semi-supervised Machine Learning Algorithms
Semi-supervised learning algorithms represent a middle ground between supervised and unsupervised algorithms. In essence, the semi-supervised model combines some aspects of both into a thing of its own.
CUSTOM AI-POWERED INFLUENCER MARKETING PLATFORM
Here’s how semi-supervised algorithms work:
- A semi-supervised machine-learning algorithm uses a limited set of labeled sample data to shape the requirements of the operation (i.e., train itself).
- The limitation results in a partially trained model that later gets the task to label the unlabeled data. Due to the limitations of the sample data set, the results are considered pseudo-labeled data.
- Finally, labeled and pseudo-labeled data sets are combined, which creates a distinct algorithm that combines descriptive and predictive aspects of supervised and unsupervised learning.
Semi-supervised learning uses the classification process to identify data assets and the clustering process to group it into distinct parts.
DATA ANALYTICS TRENDS TO PREPARE FOR A POST-PANDEMIC RESET
Semi-supervised Machine Learning Use Cases
Legal and Healthcare industries, among others, manage web content classification, image, and speech analysis with the help of semi-supervised learning.
In the case of web content classification, semi-supervised learning is applied for crawling engines and content aggregation systems. In both cases, it uses a wide array of labels to analyze content and arrange it in specific configurations. However, this procedure usually requires human input for further classification.
An excellent example of this will be uClassify. The other well-known tool of this category is the GATE (General Architecture for Text Engineering).
In the case of image and speech analysis, an algorithm performs labeling to provide a viable image or speech analytic model with coherent transcription based on a sample corpus. For example, it can be an MRI or CT scan. With a small set of exemplary scans, it is possible to provide a coherent model able to identify anomalies in the images.
ARTIFICIAL INTELLIGENCE IN ECOMMERCE: THE BEST USAGE AND TOOLS
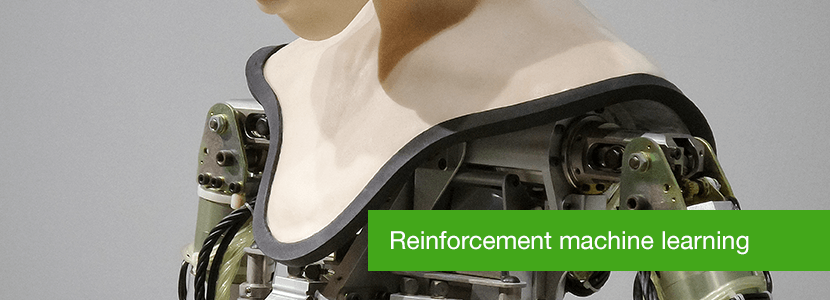
Reinforcement Learning Algorithms
Reinforcement learning represents what is commonly understood as machine learning artificial intelligence.
In essence, reinforcement learning is all about developing a self-sustained system that, throughout contiguous sequences of tries and fails, improves itself based on the combination of labeled data and interactions with the incoming data.
Reinforced ML uses the technique called exploration/exploitation. The mechanics are simple – the action takes place, the consequences are observed, and the next action considers the results of the first action.
In the center of reinforcement learning algorithms are reward signals that occur upon performing specific tasks. In a way, reward signals are serving as a navigation tool for the reinforcement algorithms. They give it an understanding of right and wrong course of action.
BEST USE CASES OF ARTIFICIAL INTELLIGENCE IN THE TRANSPORTATION INDUSTRY
Two main types of reward signals are:
- Positive reward signal encourages continuing performance a particular sequence of action
- Negative reward signal penalizes for performing certain activities and urges to correct the algorithm to stop getting penalties.
However, the function of the reward signal may vary depending on the nature of the information. Thus reward signals may be further classified depending on the requirements of the operation. Overall, the system tries to maximize positive rewards and minimize the negatives.
Most common reinforcement learning algorithms include:
- Q-Learning
- Temporal Difference (TD)
- Monte-Carlo Tree Search (MCTS)
- Asynchronous Actor-Critic Agents (A3C)
Use Cases for Reinforced Machine Learning Algorithms
Reinforcement Machine Learning fits for instances of limited or inconsistent information available. In this case, an algorithm can form its operating procedures based on interactions with data and relevant processes.
Modern NPCs and other video games use this type of machine learning model a lot. Reinforcement Learning provides flexibility to the AI reactions to the player’s action thus providing viable challenges. For example, the collision detection feature uses this type of ML algorithm for the moving vehicles and people in the Grand Theft Auto series.
Need help in developing your App?
Reach outSelf-driving cars also rely on reinforced learning algorithms as well. For example, if the self-driving car (Waymo, for instance) detects the road turn to the left – it may activate the “turn left” scenario and so on.
The most famous example of this variation of reinforcement learning is AlphaGo that went head to head with the second-best Go player in the world and outplayed him by calculating the sequences of actions out of current board position.
On the other hand, Marketing and Ad Tech operations also use Reinforcement Learning. This type of machine learning algorithm can make retargeting operation much more flexible and efficient in delivering conversion by closely adapting to the user’s behavior and surrounding context.
Also, Reinforcement learning is used to amplify and adjust natural language processing (NLP) and dialogue generation for chatbots to:
- mimic the style of an input message
- develop more engaging, informative kinds of responses
- find relevant responses according to the user reaction.
With the emergence of Google DialogFlow building, such bot became more of a UX challenge than a technical feat.
What do we think about ML intelligent algorithm?
As you can see, different types of machine learning algorithms are solving different kinds of problems. The combination of different algorithms makes a power capable of handling a wide variety of tasks and extracting valuable insights out of all sorts of information.
Whether your business is a taxi app or a food delivery service or even a social media network – every app can benefit from machine learning algorithms. Ready to begin? The APP Solutions team has expertise in architecting and implementing ML algorithms into various types of projects and we’d love to see your business grow.
I want a free consultation for my project
Contact Us